Unveiling Brain Region Patterns in PET/CT scans for Lung Cancer Assessment: A Computational AI Framework
Article Information
Hakan Sat Bozcuk1*, Ahmet Eren Sen2, Mehmet Artaç3, Bugra Kaya4
1PDepartment of Medical Oncology, Medical Park Hospital, Antalya 07230, Turkey
2Department of Nuclear Medicine, Konya City Hospital, Konya 42020, Turkey
3Department of Medical Oncology, Necmettin Erbakan University Hospital, Konya 42090 Turkey
4Department of Nuclear Medicine, Necmettin Erbakan University Hospital, Konya 42090, Turkey
*Corresponding Author: Hakan Sat Bozcuk, Department of Medical Oncology, Medical Park Hospital, Antalya 07230, Turkey.
Received: 10 September 2023; Accepted: 21 September 2023; Published: 09 October 2023
Citation: Hakan Sat Bozcuk, Ahmet Eren Sen, Mehmet Artaç, Bugra Kaya. Unveiling Brain Region Patterns in PET/CT scans for Lung Cancer Assessment: A Computational AI Framework. Journal of Cancer Science and Clinical Therapeutics. 7 (2023): 179-185.
View / Download Pdf Share at FacebookAbstract
This study aims to investigate potential differences in brain activity between lung cancer patients and healthy controls at the time of diagnosis, utilizing a computer vision artificial intelligence (AI) model. Participants undergoing evaluation for lung cancer (cases) and with benign pulmonary nodules (controls) underwent Positron Emission Tomography/ Computerized Tomography (PET/CT) scans. Specialized software reconstructed and labeled brain images. A computer vision AI model was developed using EfficientNet B0 through transfer learning, complemented by multivariate discriminant analysis. A total of 84 cases were recruited into the study. The constructed AI model exhibited robust accuracy (internal accuracy=1.0, external sensitivity=0.83) on a subset of cases (52 lung cancer patients, 22 controls). Notably, the right frontal lobe emerged as a crucial discriminator, displaying a 5% reduction in the ratio of frontal lobe to brainstem activity in lung cancer cases (Wilk’s Lambda=0.877, P=0.002). Based solely on PET/CT brain imaging data, our AI model accurately classified lung cancer patients. The distinct role of the right frontal lobe in this study underscores the broader significance, shedding light on brain function disparities at lung cancer diagnosis.
Keywords
Lung Cancer; Pet/Ct; Artificial Intelligence
Lung Cancer articles; Pet/Ct articles; Artificial Intelligence articles
Lung Cancer articles Lung Cancer Research articles Lung Cancer review articles Lung Cancer PubMed articles Lung Cancer PubMed Central articles Lung Cancer 2023 articles Lung Cancer 2024 articles Lung Cancer Scopus articles Lung Cancer impact factor journals Lung Cancer Scopus journals Lung Cancer PubMed journals Lung Cancer medical journals Lung Cancer free journals Lung Cancer best journals Lung Cancer top journals Lung Cancer free medical journals Lung Cancer famous journals Lung Cancer Google Scholar indexed journals Pet/Ct articles Pet/Ct Research articles Pet/Ct review articles Pet/Ct PubMed articles Pet/Ct PubMed Central articles Pet/Ct 2023 articles Pet/Ct 2024 articles Pet/Ct Scopus articles Pet/Ct impact factor journals Pet/Ct Scopus journals Pet/Ct PubMed journals Pet/Ct medical journals Pet/Ct free journals Pet/Ct best journals Pet/Ct top journals Pet/Ct free medical journals Pet/Ct famous journals Pet/Ct Google Scholar indexed journals Artificial Intelligence articles Artificial Intelligence Research articles Artificial Intelligence review articles Artificial Intelligence PubMed articles Artificial Intelligence PubMed Central articles Artificial Intelligence 2023 articles Artificial Intelligence 2024 articles Artificial Intelligence Scopus articles Artificial Intelligence impact factor journals Artificial Intelligence Scopus journals Artificial Intelligence PubMed journals Artificial Intelligence medical journals Artificial Intelligence free journals Artificial Intelligence best journals Artificial Intelligence top journals Artificial Intelligence free medical journals Artificial Intelligence famous journals Artificial Intelligence Google Scholar indexed journals cancer diagnosis articles cancer diagnosis Research articles cancer diagnosis review articles cancer diagnosis PubMed articles cancer diagnosis PubMed Central articles cancer diagnosis 2023 articles cancer diagnosis 2024 articles cancer diagnosis Scopus articles cancer diagnosis impact factor journals cancer diagnosis Scopus journals cancer diagnosis PubMed journals cancer diagnosis medical journals cancer diagnosis free journals cancer diagnosis best journals cancer diagnosis top journals cancer diagnosis free medical journals cancer diagnosis famous journals cancer diagnosis Google Scholar indexed journals cancer cells articles cancer cells Research articles cancer cells review articles cancer cells PubMed articles cancer cells PubMed Central articles cancer cells 2023 articles cancer cells 2024 articles cancer cells Scopus articles cancer cells impact factor journals cancer cells Scopus journals cancer cells PubMed journals cancer cells medical journals cancer cells free journals cancer cells best journals cancer cells top journals cancer cells free medical journals cancer cells famous journals cancer cells Google Scholar indexed journals malignancy articles malignancy Research articles malignancy review articles malignancy PubMed articles malignancy PubMed Central articles malignancy 2023 articles malignancy 2024 articles malignancy Scopus articles malignancy impact factor journals malignancy Scopus journals malignancy PubMed journals malignancy medical journals malignancy free journals malignancy best journals malignancy top journals malignancy free medical journals malignancy famous journals malignancy Google Scholar indexed journals therapies articles therapies Research articles therapies review articles therapies PubMed articles therapies PubMed Central articles therapies 2023 articles therapies 2024 articles therapies Scopus articles therapies impact factor journals therapies Scopus journals therapies PubMed journals therapies medical journals therapies free journals therapies best journals therapies top journals therapies free medical journals therapies famous journals therapies Google Scholar indexed journals neurological dysfunction articles neurological dysfunction Research articles neurological dysfunction review articles neurological dysfunction PubMed articles neurological dysfunction PubMed Central articles neurological dysfunction 2023 articles neurological dysfunction 2024 articles neurological dysfunction Scopus articles neurological dysfunction impact factor journals neurological dysfunction Scopus journals neurological dysfunction PubMed journals neurological dysfunction medical journals neurological dysfunction free journals neurological dysfunction best journals neurological dysfunction top journals neurological dysfunction free medical journals neurological dysfunction famous journals neurological dysfunction Google Scholar indexed journals cranial metastasis articles cranial metastasis Research articles cranial metastasis review articles cranial metastasis PubMed articles cranial metastasis PubMed Central articles cranial metastasis 2023 articles cranial metastasis 2024 articles cranial metastasis Scopus articles cranial metastasis impact factor journals cranial metastasis Scopus journals cranial metastasis PubMed journals cranial metastasis medical journals cranial metastasis free journals cranial metastasis best journals cranial metastasis top journals cranial metastasis free medical journals cranial metastasis famous journals cranial metastasis Google Scholar indexed journals Oncology articles Oncology Research articles Oncology review articles Oncology PubMed articles Oncology PubMed Central articles Oncology 2023 articles Oncology 2024 articles Oncology Scopus articles Oncology impact factor journals Oncology Scopus journals Oncology PubMed journals Oncology medical journals Oncology free journals Oncology best journals Oncology top journals Oncology free medical journals Oncology famous journals Oncology Google Scholar indexed journals
Article Details
1. Introduction
The intricate communication between cancer cells and infiltrating neural cells is acknowledged [1]. However, it remains unclear whether such a connection exists between cancer cells and brain neural cells. An extensive body of literature highlights the potential impact of cancer treatments and associated conditions on the brain function of cancer patients. Yet, the precise relationship between brain functions and cancer development during the diagnostic phase remains elusive [2, 3]. Theoretically, given that cancer development and progression disrupt and modify the mechanisms governing nervous system development and regeneration, the nervous system could play a pivotal role in various aspects of cancer. Moreover, the reciprocal relationship between cancer and its therapies, with the potential to reshape the nervous system, introduces the possibility of neurological dysfunction or even malignancy development [4]. Conversely, it is plausible that brain dysfunction might contribute to the progression of cancer. Consequently, investigating the presence of any brain dysfunction at the time of cancer diagnosis, even prior to the initiation of treatment and any potential treatment-induced brain damage, holds significant importance in order to enhance our understanding of the intricate association between brain functions and cancer development. Artificial intelligence (AI) models for image classification have demonstrated remarkable success in various medical contexts, including the assessment of brain tumors via magnetic resonance imaging (MRI), analysis of blood leucocyte images, diverse medical imaging modalities, lung computed tomography (CT) scans, skin lesion evaluations, and breast cancer histological images [5-11]. While Positron Emission Tomography / Computerized Tomography (PET / CT) scans are widely utilized in oncology to monitor cancer occurrence and progression, their sensitivity in detecting cranial metastasis remains limited. In contrast, PET scans have proven valuable in elucidating the functions of brain centers in various conditions, including depression [12, 13]. A plethora of pretrained computer vision models, such as Efficient-Net, Inception, ResNet, MobileNet, NasNet, and VGG, are available. Fine- tuning these models with additional training images has been shown to enhance their accuracy [14-20]. These models, once suitably trained, can be harnessed for diverse tasks, spanning from medical diagnostics to general applications like facial recognition, road lane detection, and vehicle license plate recognition [21]. Accordingly, our study follows a similar methodology. In light of these considerations, our study endeavors to leverage artificial intelligence techniques to detect discernible patterns of brain activity in patients diagnosed with lung cancer and healthy controls at the point of diagnosis. Furthermore, we delve into identifying the brain centers that wield the greatest significance in the intricate interplay between brain function and cancer development.
2. Methods and Materials
2.1 Recruitment of Patients and Controls
Participants diagnosed with lung cancer, with or without a confirmed pathological diagnosis, and who had undergone PET/CT scans at the time of diagnosis, were enrolled in the study. Both Non-Small Cell Lung Cancer (NSCLC) and Small Cell Lung Cancer (SCLC) pathologies were considered. Additionally, patients with benign lung nodules undergoing diagnostic evaluation were included. Both lung cancer patients and healthy controls with benign nodules were assessed at Necmettin Erbakan University's Medical Oncology and Nuclear Medicine Departments. Data spanning from September 20, 2022, to April 21, 2023, were retrieved from the hospital image database. Exclusion criteria encompassed cerebral metastatic disease on PET/CT, conditions impacting brain function (e.g., active psychiatric illness, addiction, organ failure), history of cerebrovascular events or brain surgery, and uncontrolled Diabetes Mellitus. Ethical approval was obtained from the Necmettin Erbakan University Ethical Committee.
2.2 Brain PET/CT Image Reconstruction
Routine PET/CT imaging was performed using F18-FDG doses ranging from 7 to 12 mCi on the Siemens Biograph 6 True Point PET/BT scanner. The Syngo Via software and MI neurology program, incorporating automated anatomical labeling, were employed [22]. This facilitated calculation of specific SUV mean outputs for various brain regions, including total brain, brain stem, bilateral frontal lobes, and multiple other regions (inferior frontal gyrus opercular, orbital, triangular, middle frontal gyrus and orbital area, superior frontal gyrus orbital, medial orbital, medial and dorsolateral area, supplementary motor area, precentral gyrus, temporal lobe, superior, middle, inferior temporal gyri, insula, fusiform gyrus, hippocampus, parahippocampus, amygdala, parietal lobe, superior parietal gyrus, supramarginal and angular gyri, inferior parietal gyrus (except supramarginal and angular gyri), postcentral gyrus, cerebellar hemi-spheres, thalamus, caudate nucleus, putamen, pallidum, cingulate and paracingulate gyri).
2.3 Development and Testing of Computer Vision AI Model
The EfficientNet B0 pretrained model was fine-tuned in Google Collab Pro Medium via transfer learning. This process involved appending a sequential dense layer to the feature extractor, followed by a final dense layer with sigmoid activation for classification [14]. While other models like EfficientNet B3 and B7, Inception V3, ResNet V2, and MobileNet V2 were considered, EfficientNet B0 demonstrated superior accuracy during experimentation. The model was trained for 12 epochs, utilizing a learning rate of 0.001 and binary cross-entropy as the loss function. Post-training, the model's accuracy and loss curves were visualized using the Matplotlib library [23]. Additional libraries, including NumPy, TensorFlow, and Keras, were employed for model development [24-26]. To enhance the pretrained model's performance, sequential dense layers were introduced, augmenting the neural network. These layers connect each neuron (or unit) to all neurons in the preceding layer [27]. For a clearer understanding of the computer vision AI algorithm's functionality, regions of focus within brain PET/CT images were depicted using heatmaps and explainable artificial intelligence (XAI) views, facilitated by the Why library [28].
2.4 Discriminant Analysis of Brain Functions
Exploration of brain regions potentially linked to lung cancer development commenced with univariate discriminant analysis. Given the numerous brain regions and their functions (both raw and as a ratio of brain stem activity), univariate tests were iterated around 200 times. A significance threshold of P < 0.20 guided the selection of features for inclusion in the multivariate stage. Bonferroni correction, employing a P value of 0.20/200, mandated a modified significance level of 0.001 or lower for the univariate tests [29]. In the multivariate discriminant analysis, significance was determined using tolerance and Wilk’s Lambda, with a P value < 0.05 considered statistically significant.
3. Results
3.1 General Characteristics:
The study encompassed a total of 84 participants (57 lung cancer patients and 27 healthy controls). The construction of the computer vision AI model utilized data from 74 cases with technically sufficient reconstructed brain image data (52 lung cancer patients and 22 healthy controls). The median age of the entire cohort was 62.5, with 80% of the cases representing male participants. Among the lung cancer group, the prevailing pathology observed was Squamous Non-Small Cell Lung Cancer (NSCLC), accounting for 51% of cases. For details, please refer to Table 1.
Table 1: Patient and Healthy Control Characteristics
Lung Cancer |
Heathy |
Total |
|
N (%) |
57 (68) |
27 (32) |
84 (100) |
Age (Median (Min-Max)) |
63 (47-85) |
61 (24-75) |
62.5 (24-85) |
Gender |
|||
Male (%) |
90 |
59 |
80 |
Female (%) |
10 |
41 |
20 |
Pathology |
|||
Squamous NSCLC (%) |
51 |
n/e |
n/e |
Non-Squamous NSCLC (%) |
23 |
n/e |
n/e |
Small Cell Lung Cancer (%) |
16 |
n/e |
n/e |
n/e; not eligible
3.2 Computer Vision Artificial Intelligence Model
The AI model was rooted in the pretrained EfficientNet B0 model, refined through fine-tuning using multiple brain/CT images from each of the 74 cases. In addition to the feature extractor layer, two sequential dense neural network layers were integrated into the model. A succinct summary of the model's architecture is available in Table 2. The compilation of AI model images from each case encompassed axial, coronal, and sagittal views, along with right lateral, left lateral, anterior, posterior, superior, inferior, right medial, and left medial views, culminating in a total of 11 views per case. For a visual understanding of the image types employed in the model, please consult Figure 1.
![]() |
Table 2: Computer vision Artificial Intelligence Model Summary
Figure 1a showcases the baseline images, while Figure 1b illustrates the heat map view, delineating crucial areas for classification. Furthermore, Figure 1c offers an example of the explainable artificial intelligence (XAI) view, highlighting pivotal regions for the AI model's construction. Following 12 training rounds (epochs), the model achieved an accuracy of 1.00 and a loss of 0.14. However, within the validation set, which constituted 20% of the training dataset, accuracy and loss figures stood at 0.64 and 3.26, respectively. Comprehensive insights into the AI model's training and loss curves are elucidated in Figure 2. Employing a distinct set of brain images from six lung cancer patients and healthy controls, the model accurately predicted the labels for five cases (sensitivity=0.83).

Figure 1: PET/CT scans findings for the brain regions in lung cancer cases and controls
3.3 Discriminant Analysis of Brain Functions between Lung Cancer Patients and Healthy Controls
Accounting for the Bonferroni correction-defined significance thresh-old of P ≤ 0.001, the univariate discriminant analysis unveiled four significant brain regions. These encompassed the right frontal lobe, left frontal lobe, right frontal opercular area, and right middle frontal gyrus. Additionally, gender emerged as the fifth significant feature within the univariate analysis. Adopting a stepwise approach in multivariate discriminant analysis, solely the right frontal lobe and gender retained their significance out of the four brain regions and gender. For the right frontal lobe, the P value stood at 0.002, Wilk’s Lambda registered at 0.877, and the tolerance figure reported as 0.955. For comprehensive insights into the discriminant analysis, consult Table 3, while significant associations within the univariate and multivariate tests are expounded in Figure 3 and Figure 4.
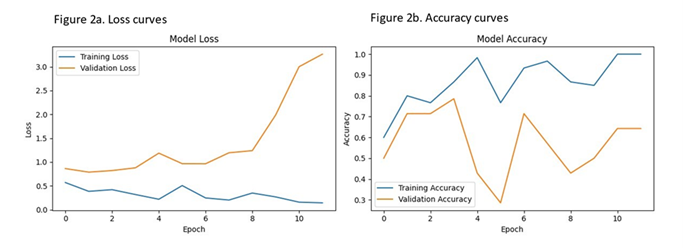
Figure 2: Loss and accuracy curves of the computer vision model
Table 3: Analysis of patient features and brain regions with significant activity in cases with lung cancer and healthy controls
Univariate Discriminant Analysis |
Multivariate Discriminant Analysis |
||||||
Feature or Brain Region |
Wilk's Lambda |
Chi-square |
df |
P value |
Tolerance |
Wilk's Lambda |
P value |
Gender |
0.877 |
10.729 |
1 |
0.001 |
0.995 |
0.864 |
0.005* |
Right Frontal Lobe |
0.864 |
11.932 |
1 |
0.001 |
0.995 |
0.877 |
0.002* |
Left Frontal Lobe |
0.883 |
10.177 |
1 |
0.001 |
0.6 |
0.775 |
0.376 |
Right Frontal Opercular Area |
0.874 |
10.981 |
1 |
0.001 |
0.736 |
0.746 |
0.05 |
Right Middle Frontal Gyrus |
0.869 |
11.454 |
1 |
0.001 |
0.403 |
0.765 |
0.186 |
*; Selected by the stepwise method as statistically significant |
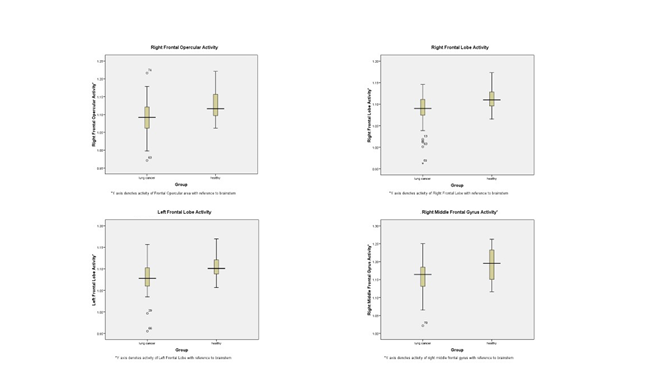
Figure 3: Activity of selected brain regions on PET/CT with and without lung cancer
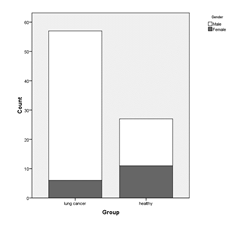
Figure 4: Gender Distribution
4. Discussion
Our study substantiates the presence of functional discrepancies within specific brain regions, as evidenced by PET/CT evaluations, in lung cancer patients at the point of diagnosis, even before any therapeutic interventions are initiated. Interestingly, through a focused analysis of concealed brain functions, our AI model effectively distinguishes between lung cancer patients and healthy controls. This finding is poised to illuminate the intricate connections between the nervous system and cancer. The exploration of whether this communication is facilitated or amplified by the immune system, as observed in the neuro immune oncology framework, and the potential temporal relationship between changes in brain function and cancer development stand as pivotal questions that warrant further investigation. A key contributor to the distinct functional profiles of brain regions in lung cancer patients and healthy controls could potentially be linked to the hypoactivity of the right frontal lobe. Our findings revealed a lower right frontal to brainstem activity ratio in lung cancer patients compared to healthy controls. The right frontal lobe holds significant functions; while the right hemisphere plays a role in non-verbal abilities, the left hemisphere is involved in controlling language-related movement. Notably, frontal lobe damage frequently manifests as challenges in processing environmental feedback. This encompasses behaviors such as perseveration, risk-taking, non-compliance with rules, and impaired associative learning—all of which can stem from frontal lobe dysfunction [30]. Exploring whether these difficulties are more prevalent in the cancer population compared to healthy controls during cancer diagnosis is an avenue worth investigating. Furthermore, discerning whether these behavioral and neurological changes merely accompany the cancer development process or play an active role in influencing or being influenced by cancer development is of interest. The evolution of medicine recently is undeniably intertwined with the rise of AI [31]. Our study underscores the feasibility of constructing robust AI models utilizing visual data from PET/CT brain images. To our knowledge, this is one of the pioneering instances of a correlative AI study within the cancer population utilizing brain PET/CT data. Other AI investigations focusing on the brain have primarily employed different imaging modalities, such as brain MRI, to address varied needs. For example, the synergy of AI technology has notably enhanced the utility of MRI imaging [32]. However, our study underscores the potential of PET/CT brain images to offer critical insights into brain region functionality in cancer patients. While we believe our findings present significant contributions, limitations exist, with the most notable being the relatively modest sample size. Recognizing that computer vision AI models thrive with larger sample sizes, we acknowledge the possibility of missing certain patterns in our model [33]. Moreover, external images are imperative to fully gauge the utility of the model. Diversifying our patient pool to encompass other common cancers could shed light on whether the functional patterns observed in our brain regions are universally applicable. Notwithstanding these limitations, we believe that our findings hold sufficient strength to spur the formulation of novel hypotheses. In essence, our study demonstrates that lung cancer patients’ manifest distinct patterns of brain function compared to healthy controls, discernible at the time of diagnosis. Notably, the activity of the right frontal lobe emerges as a pivotal factor in this divergence. The ability of our AI model to accurately categorize cases within the lung cancer group or healthy controls underscores its clinical potential. Yet, further investigations are imperative to fully elucidate the interplay between the nervous system and cancer in our findings.
Conclusions
At the time of diagnosis, before receiving any treatment, lung cancer patients have a different brain function pattern that is identifiable by an artificial intelligence model. The diminished right frontal lobe activity may be one of the fundamental components of this different brain function pattern seen in lung cancer patients.
Author Contributions
Hakan Sat Bozcuk; found the study question, designed the study, analysed the study, wrote the paper. Ahmet Eren Sen; Collected the data, revised the paper. Mehmet Artaç co designed the study, revised the paper. Bugra Kaya; Participated in data collection, also revised the paper.
Funding
This research received no external funding.
Institutional Review Board Statement
The study was conducted in accordance with the Declaration of Helsinki, and approved by the or Ethics Committee of Necmettin Erbakan University in 2022.
Informed Consent Statement
“Informed consent was obtained from all subjects involved in the study”.
Written informed consent for publication must be obtained from participating patients who can be identified (including by the patients themselves). Please state “Written informed consent has been obtained from the patient(s) to publish this paper” if applicable.
Data Availability Statement
Data is unavailable due to privacy or ethical restrictions. Unidentifiable numerical patient data in tabular format is available upon request.
Acknowledgments
We thank Levent Çapan, for being an inspiration for the study.
Conflicts of Interest
The authors declare no conflict of interest.
References
- Silverman DA, Martinez VK, Dougherty PM, et al. Cancer-Associated Neurogenesis and Nerve-Cancer Cross-talk. Cancer Res 81 (2021): 1431-1440.
- Kesler SR, Sleurs C, McDonald BC, et al. Brain imaging in pediatric cancer survivors: correlates of cognitive impairment. J Clin Oncol 39 (2021): 1775–1785.
- McDonald BC. Structural neuroimaging findings related to adult non-CNS cancer and treatment: Review, integration, and implications for treatment of cognitive dysfunction: Review. Neurotherapeutics 18 (2021): 792-810.
- Winkler F, Venkatesh HS, Amit M, et al. Cancer neuroscience: State of the field, emerging directions. Cell 186 (2023): 1689-1707.
- Sultan HH, Nancy MS, Al-Atabany W. Multi-Classification of Brain Tumor Images Using Deep Neural Network. IEEE Access 7 (2019): 69215-69225.
- Anaraki AK, Ayati M, Kazemi F. Magnetic resonance imaging-based brain tumor grades classification and grading via convolutional neural networks and genetic algorithms. Biocybernetics and Biomedica Engineering 39 (2019): 63-74.
- Hasan AM, Jalab HA, Meziane F, et al. Combining Deep and Handcrafted Image Features for MRI Brain Scan Classification. IEEE Access 7 (2019): 79959-79967.
- Liang G, Hong H, Xie W, et al. Combining Convolutional Neural Network with Recursive Neural Network for Blood Cell image classification. IEEE Access 6 (2018): 36188-36197.
- Srivastava V, Purwar RK. Classification of CT Scan Images of Lungs Using Deep Convolutional Neural Network with External Shape-Based Features. J Digit Imaging 33 (2020): 252-261.
- Mahbod A, Schaefer G, Wang C, et al. Skin Lesion Classification Using Hybrid Deep Neural Networks. ICASSP (2019).
- Anupama MA, Sowmya V, Soman K. Breast Cancer Classification using Capsule Network with Preprocessed Histology Images. ICCSP (2019).
- Chan TK, Li WI, Lau JHYH, et al. The diagnostic performance of 18 fluorine-fluorodeoxyglucose PET for brain metastases: a systematic review and meta-analysis. Nucl Med Commun 44 (2023): 741-749.
- Sankar A, Melin A, Lorenzetti V, et al. A systematic review and meta-analysis of the neural correlates of psychological therapies in major depression. Psychiatry Res Neuroimaging 279 (2018): 31-39.
- Tan M, Le QV. EfficientNet: Rethinking Model Scaling for Convolutional Neural Networks (2020). DOI: https://doi.org/10.48550/arXiv.1905.11946.
- Szegedy C, Liu W, Jia Y, et al. Going Deeper with Convolutions (2014). arXiv:1409.4842 2014; DOI: https://doi.org/10.48550/arXiv.1409.4842.
- He K, Zhang X, Ren S, et al. Deep Residual Learning for Image Recognition. CVPR (2016). DOI: 10.1109/CVPR.2016.90.
- Howard AG, Zhu M, Chen B, et al. MobileNets: efficient convolutional neural networks for mobile vision applications (2017). arXiv:1704.04861 2017; DOI: https://doi.org/10.48550/arXiv.1704.04861.
- Zoph B, Vasudevan V, Shlens J, et al. Learning Transferable Architectures for Scalable Image Recognition (2023). arXiv:1707.07012v4 2018; at https://arxiv.org/pdf/1707.07012.pdf. Accessed at 23.7.2023.
- Simonyan K, Zisserman A. Very Deep Convolutional Networks for large-scale image recognition 6 (2015). arXiv:1409.1556v6 2015; DOI: https://doi.org/10.48550/arXiv.1409.1556.
- Model fine tuning (2023). https://clemsonciti.github.io/rcde_workshops/pytorch_advanced/03html. Accessed at 8/18/2023.
- 15 Computer Visions Projects You Can Do Right Now (2023). https://neptune.ai/blog/15- computer-visions-projects. Accessed at 8/18/2023
- Fahmi R, Platsch G. Syngo.via database comparison in MI Neurology (2023). https://www.siemens-healthineers.com/tr/molecular-imaging/mi-clinical- corner/whitepapers/syngo-via-database-comparison-in-mi-neurology. Accessed at 8/19/2023.
- Matplotlib: Visualization with Python (2023). https://matplotlib.org. Accessed at 8/19/2023
- NumPy (2023). https://numpy.org. Accessed at 8/19/2023
- Create production-grade machine learning models with TensorFlow (2023). https://www.tensorflow.org. Accessed at 8/19/2023
- Keras (2023). https://keras.io. Accessed at 8/19/2023
- Verma Y. A complete understanding of dense layers in neural networks (2023). https://analyticsindiamag.com/a-complete-understanding-of-dense-layers-in-neural-networks. Accessed at 8/19/2023.
- Sayici MB. Why. Framework-agnostic XAI Library for Computer Vision, for understanding why models behave that way (2023). https://github.com/mburaksayici/Why. Accessed at 8/19/2023.
- The Bonferroni Correction Method Explained (2023). https://toptipbio.com/bonferroni-correction-method/#google_vignette. Accessed at 8/19/2023.
- Centre for neuro skills. Frontal lobes (2023). https://www.neuroskills.com/brain-injury/frontal-lobes/#:~:text=The%20left%20frontal%20lobe%20is,involved%20in%20nearly%20all%20behavior. Accessed at 8/19/2023.
- Beam AL, Drazen JM, Kohane IS, et al. Artificial Intelligence in Medicine. N Engl J Med 388 (2023): 1220-1221.
- Bria A. Taking MRI to the next level of care with AI (2023). https://blog.medicai.io/en/taking-mri-to-the-next-level-of-care-with-ai. Accessed at 8/19/2023.
- Rajput D, Wang WJ, Chen CC. Evaluation of a decided sample size in machine learning applications. BMC Bioinformatics 24 (2023): 48.