Supervised Machine Learning Techniques For the Prediction of Hepatocellular Carcinoma Recurrence
Article Information
Andrea Mega1*, Luca Marzi1, Alessandra Andreotti2, Fabio Vittadello2, Filippo Pelizzaro3,
Stefano Gitto4, Gilbert Spizzo5,6, Federica Ferro7, Antonio Frena8, Andreas Seeber5
1Department of Gastroenterology, San Maurizio Regional Hospital, Bolzano, Italy
2Explora Research and Statistical Analysis, Padova, Italy
3Gastroenterology Unit, Department of Surgery, Oncology and Gastroenterology, University of Padova, Padova, Italy
4Department of Experimental and Clinical Medicine, University of Florence, Italy
5Department of Hematology and Oncology, Comprehensive Cancer Center Innsbruck, Medical University of Innsbruck, Innsbruck, Austria
6Department of Internal Medicine, Hospital of Bressanone, Bressanone, Italy
7Department of Radiology, San Maurizio Regional Hospital, Bolzano, Italy
8Department of Surgery, San Maurizio Regional Hospital, Bolzano, Italy
*Corresponding Author: Andrea Mega, Department of Gastroenterology, San Maurizio Regional Hospital, Bolzano, Italy
Received: 22 March 2022; Accepted: 29 March 2022; Published: 27 April 2022
Citation: Andrea Mega, Luca Marzi, Alessandra Andreotti, Fabio Vittadello, Filippo Pelizzaro, Stefano Gitto, Gilbert Spizzo, Federica Ferro, Antonio Frena, Andreas Seeber. Supervised Machine Learning Techniques For the Prediction of Hepatocellular Carcinoma Recurrence. Journal of Surgery and Research 5 (2022): 238-251.
View / Download Pdf Share at FacebookAbstract
Background & Aims
Hepatocellular carcinoma (HCC) is the most frequent malignant tumor of the liver and its incidence is increasing worldwide. Several treatments are currently available, but predictors of cancer recurrence are poorly characterized. The development of artificial intelligence has recently made available a new tool called Machine Learning (ML). ML allows running strong prediction of several variables, after inputting several data into a dedicated software. This study aimed to create a MLmodel for predicting HCC recurrence.
Patients and methods
In this study, we analyzed retrospectively data of 166 patients who were managed at the Bolzano Regional Hospital between 1998 and 2019. In order to find the best predictive model, either both non-parametric and parametric models were evaluated. Non-parametric models trained in this study were the following: Random Forest (RF), Support Vector Machine (SVM) and K-Nearest Neighbours (KNN). Parametric model adopted was the logistic regression model with the elastic net algorithm (ENET).
Results
In our dataset, the Random Forest model is the most performant (AUC 0.712). Independently from the treatment performed, age at diagnosis, MELD, the absence of previous obesity, type of diagnosis, BMI, and BCLC emerged as significant HCC recurrence predictors.
Conclusion
ML may be a valuable tool in the prediction of HCC recurrence. Larger sample sizes are needed to create useful tool for the clinical management of patients with HCC.
Keywords
Hepatocellular carcinoma, Supervised Machine Learning, Artificial Intelligence, Prediction of recurrence
Hepatocellular carcinoma articles; Supervised Machine Learning articles; Artificial Intelligence articles; Prediction of recurrence articles
Hepatocellular carcinoma articles Hepatocellular carcinoma Research articles Hepatocellular carcinoma review articles Hepatocellular carcinoma PubMed articles Hepatocellular carcinoma PubMed Central articles Hepatocellular carcinoma 2023 articles Hepatocellular carcinoma 2024 articles Hepatocellular carcinoma Scopus articles Hepatocellular carcinoma impact factor journals Hepatocellular carcinoma Scopus journals Hepatocellular carcinoma PubMed journals Hepatocellular carcinoma medical journals Hepatocellular carcinoma free journals Hepatocellular carcinoma best journals Hepatocellular carcinoma top journals Hepatocellular carcinoma free medical journals Hepatocellular carcinoma famous journals Hepatocellular carcinoma Google Scholar indexed journals Supervised Machine Learning articles Supervised Machine Learning Research articles Supervised Machine Learning review articles Supervised Machine Learning PubMed articles Supervised Machine Learning PubMed Central articles Supervised Machine Learning 2023 articles Supervised Machine Learning 2024 articles Supervised Machine Learning Scopus articles Supervised Machine Learning impact factor journals Supervised Machine Learning Scopus journals Supervised Machine Learning PubMed journals Supervised Machine Learning medical journals Supervised Machine Learning free journals Supervised Machine Learning best journals Supervised Machine Learning top journals Supervised Machine Learning free medical journals Supervised Machine Learning famous journals Supervised Machine Learning Google Scholar indexed journals Artificial Intelligence articles Artificial Intelligence Research articles Artificial Intelligence review articles Artificial Intelligence PubMed articles Artificial Intelligence PubMed Central articles Artificial Intelligence 2023 articles Artificial Intelligence 2024 articles Artificial Intelligence Scopus articles Artificial Intelligence impact factor journals Artificial Intelligence Scopus journals Artificial Intelligence PubMed journals Artificial Intelligence medical journals Artificial Intelligence free journals Artificial Intelligence best journals Artificial Intelligence top journals Artificial Intelligence free medical journals Artificial Intelligence famous journals Artificial Intelligence Google Scholar indexed journals Prediction of recurrence articles Prediction of recurrence Research articles Prediction of recurrence review articles Prediction of recurrence PubMed articles Prediction of recurrence PubMed Central articles Prediction of recurrence 2023 articles Prediction of recurrence 2024 articles Prediction of recurrence Scopus articles Prediction of recurrence impact factor journals Prediction of recurrence Scopus journals Prediction of recurrence PubMed journals Prediction of recurrence medical journals Prediction of recurrence free journals Prediction of recurrence best journals Prediction of recurrence top journals Prediction of recurrence free medical journals Prediction of recurrence famous journals Prediction of recurrence Google Scholar indexed journals surgical resection articles surgical resection Research articles surgical resection review articles surgical resection PubMed articles surgical resection PubMed Central articles surgical resection 2023 articles surgical resection 2024 articles surgical resection Scopus articles surgical resection impact factor journals surgical resection Scopus journals surgical resection PubMed journals surgical resection medical journals surgical resection free journals surgical resection best journals surgical resection top journals surgical resection free medical journals surgical resection famous journals surgical resection Google Scholar indexed journals orthotopic liver transplantation articles orthotopic liver transplantation Research articles orthotopic liver transplantation review articles orthotopic liver transplantation PubMed articles orthotopic liver transplantation PubMed Central articles orthotopic liver transplantation 2023 articles orthotopic liver transplantation 2024 articles orthotopic liver transplantation Scopus articles orthotopic liver transplantation impact factor journals orthotopic liver transplantation Scopus journals orthotopic liver transplantation PubMed journals orthotopic liver transplantation medical journals orthotopic liver transplantation free journals orthotopic liver transplantation best journals orthotopic liver transplantation top journals orthotopic liver transplantation free medical journals orthotopic liver transplantation famous journals orthotopic liver transplantation Google Scholar indexed journals magnetic resonance imaging articles magnetic resonance imaging Research articles magnetic resonance imaging review articles magnetic resonance imaging PubMed articles magnetic resonance imaging PubMed Central articles magnetic resonance imaging 2023 articles magnetic resonance imaging 2024 articles magnetic resonance imaging Scopus articles magnetic resonance imaging impact factor journals magnetic resonance imaging Scopus journals magnetic resonance imaging PubMed journals magnetic resonance imaging medical journals magnetic resonance imaging free journals magnetic resonance imaging best journals magnetic resonance imaging top journals magnetic resonance imaging free medical journals magnetic resonance imaging famous journals magnetic resonance imaging Google Scholar indexed journals Supervised Machine Learning techniques articles Supervised Machine Learning techniques Research articles Supervised Machine Learning techniques review articles Supervised Machine Learning techniques PubMed articles Supervised Machine Learning techniques PubMed Central articles Supervised Machine Learning techniques 2023 articles Supervised Machine Learning techniques 2024 articles Supervised Machine Learning techniques Scopus articles Supervised Machine Learning techniques impact factor journals Supervised Machine Learning techniques Scopus journals Supervised Machine Learning techniques PubMed journals Supervised Machine Learning techniques medical journals Supervised Machine Learning techniques free journals Supervised Machine Learning techniques best journals Supervised Machine Learning techniques top journals Supervised Machine Learning techniques free medical journals Supervised Machine Learning techniques famous journals Supervised Machine Learning techniques Google Scholar indexed journals Italian Liver Cancer articles Italian Liver Cancer Research articles Italian Liver Cancer review articles Italian Liver Cancer PubMed articles Italian Liver Cancer PubMed Central articles Italian Liver Cancer 2023 articles Italian Liver Cancer 2024 articles Italian Liver Cancer Scopus articles Italian Liver Cancer impact factor journals Italian Liver Cancer Scopus journals Italian Liver Cancer PubMed journals Italian Liver Cancer medical journals Italian Liver Cancer free journals Italian Liver Cancer best journals Italian Liver Cancer top journals Italian Liver Cancer free medical journals Italian Liver Cancer famous journals Italian Liver Cancer Google Scholar indexed journals
Article Details
1. Introduction
Hepatocellular carcinoma (HCC) is the sixth most common cancer and the fourth leading cause of cancer-related death worldwide [1]. Despite the eradication of Hepatitis C Virus (HCV) and the available vaccination for Hepatitis B Virus (HBV), the incidence of HCC is predicted to increase in the coming years, due to population growth, aging and the expansion of Nonalcoholic Fatty Liver Disease/ Nonalcoholic Steatohepatitis (NAFLD/NASH) in Western countries [2]. In HCC, the prediction of prognosis is complex since not only tumor burden, but also residual liver function and general clinical conditions (e.g., performance status) must be take into account. Furthermore, ongoing treatment represents one of the most important prognostic parameters [3]. Despite noteworthy improvements in screening and treatment, the recurrence and/or progression rate and the cancer-related mortality rate remains high [42]. HCC has a high recurrence rate after both surgical resection and orthotopic liver transplantation. In particular, after a liver transplantation patient show a five-year survival rate of 65-81% despite using specific criteria (Milan, USFC, Kyoto) aimed at selecting patients thought to have better long-term outcomes [4,5]. Conventional predictive models to assess the prognosis of HCC include survival analysis, logistic regression, and Cox models [6,7]. These statistical models are usually based on multivariate predictors, such as demographic, clinical, radiological, pathological, and genetic parameters. However, considered the extremely complex prognostic prediction of HCC patients, linear systems might be too simplistic [8]. Artificial intelligence (AI) is a very promising tool that may be useful in overcoming the limitations of traditional statistic and that can improve the prediction of survival and recurrence after treatment [43]. AI refers to the use of computers and related technologies to simulate the intelligent behavior and critical thinking of humans [9]. Although AI has been applied in medicine for the past 30 years, only recent studies demonstrated the ability of this tool to achieve accurate diagnosis and tumor classification, as well as the evaluation of survival and recurrence [44]. Among AI technologies, machine learning (ML) relies on the computer’s ability to “learn” and improve from past examples without being programmed [45]. Deep learning (DL) is a subset of ML and a computer software that mimics the network of neurons in a brain, where the learning phase occurs through a neural network. Since ML models can include a lot of variables, it is advantageous and can become a promising tool compared to the traditional statistical models [45]. Consequently, with the introduction of AI several computer models have been developed for the prediction of tumor characteristics or of recurrence risk, using ultrasound-based radiomic signatures [10], contrast-enhanced computed tomography (CT) [11-13] or magnetic resonance imaging (MRI) [14]. Other authors have also developed predictive models in patients undergoing radiofrequency ablation (RFA) based on Support Vector Machine (SVM) [15]. Algorithms have been created using the combination of CT images and clinical data to predict response to transarterial chemoembolization (TACE) [16], DL models using CT or ultrasound images [17,18], ML techniques by combining MRI with clinical data [19], or SVM techniques to genetic analysis [20]. To date, algorithms have been developed to predict the response and survival after TACE, stereotaxic body radiotherapy (SBRT), RFA and liver resection. In addition, AI techniques were evaluated to providing information on the predictive power of biomarkers [21-34]. The aim of this study was to create a predictive model for elaboration the recurrence of HCC after treatment, and to identify the most important clinical features associated with disease recurrence, regardless of the type of treatment, using Supervised Machine Learning techniques.
2. Materials and Methods
2.1 Cohort description
Data were retrieved from the Italian Liver Cancer (ITA.LI.CA.) database, a national registry that prospectively collect the data of patients with HCC managed in 20 Italian centers. Currently, the database includes 171 patients diagnosed with HCC between January 1998 and December 2018. Informed consent was obtained as usual for medical, surgical, and radiological treatments, but not specifically for patient data to be used in this retrospective study. Details about patient data collected for this study are described in S1.
2.2 Machine Learning Models
For the training of ML models and subsequent accuracy evaluation, the dataset was randomly divided in a training/validation set (70% of cases) and a test set (30% of cases). 5-fold cross-validation with 20 repetitions was applied. Considered that the Receiver Operating Characteristic (ROC) curve is more robust than classical accuracy, especially in the case of unbalanced outcomes [35], it was used as metric in the training process. In this study, both non-parametric models and parametric models have been evaluated. The nonparametric models trained were the following: Random Forest, Support Vector Machine and K-Nearest Neighbours. As parametric model, we used the logistic regression model with the elastic net algorithm [36]. A disparity in the frequencies of the observed classes can have a significant negative impact on the fit of the model. To solve this problem, we used the "up-sampling" method [37], which randomly samples (with replacement) the minority class (absence of relapse) to be of the same size as the majority class (presence of relapse) (Figure 1).
Therefore, the metrics used in this study are the following:
- Balanced precision: the quality of a positive prediction made by the model. Precision refers to the number of true positives divided by the total number of positive predictions (i.e., the number of true positives plus the number of false positives).
- Balanced recall: the ability of a model to find all the relevant cases within a data set. Recall refers to the number of true positives divided by the number of true positives plus the number of false negatives. In binary classification, recall is called sensitivity or true positive rate.
- Balanced accuracy: the percentage of correct predictions for the test data.
- F1 balanced metric: The F1 score is the 2*((precision*recall)/(precision+recall)). It is also called the F Score or the F Measure. The F1 score conveys the balance between precision and recall.
Having used the ROC metric in training the model, the optimal cut-off was appropriately investigated, and the performance metrics have been calculated using this cut-off (and not the default one equal to 0.5) [38].
The statistical analyses have been performed using the caret package in R, version 4.1.0 [39].
3. Results
3.1 Characteristics of the population used for Model Building
For the present study, the 171 patients managed at the Bolzano Regional Hospital from 1998 and 2019 were analyzed. After the exclusion of 5 patients without complete data, the final dataset was composed of 166 patients. Medical examinations were conducted between 1998 and 2020. The number of medical examinations for each patient varies from a minimum of 1 to a maximum of 10. The median follow-up was 520.5 (range, 23 days – 11.8 years). About 2/3 of the patients (65.7%) showed at least one recurrence during the study observation and therefore the outcome variable is unbalanced. Demographic and clinical characteristics of patients included in this study are described in table 1.
Socio-demographic variables |
|
Female gender, No (%) |
15 (9.0) |
Age at diagnosis (years) |
67.9 (9.27) |
HCC diagnosis |
|
Type of HCC diagnosis, No (%) |
|
Unknown |
1 (0.6) |
Surveillance |
62 (37.0) |
Causal |
73 (44.0) |
Symptomatic |
30 (18.1) |
Patient's medical history |
|
Alcohol, No (%) |
|
No |
53 (31.9) |
Yes |
109 (65.7) |
not evaluated |
4 (2.4) |
HBsAg, No (%) |
|
Negative |
152 (91.6) |
Positive |
14 (8.4) |
anti-HCV, No (%) |
|
Negative |
123 (74.1) |
Positive |
43 (25.9) |
Comorbidities |
|
BMI, No (%) |
|
not known |
25 (15.1) |
Underweight |
1 (0.6) |
normal weight |
52 (31.3) |
Overweight |
69 (41.6) |
Obesity |
19 (11.4) |
Previous obesity (BMI> 30), No (%) |
|
No |
107 (64.5) |
Yes |
21 (12.7) |
not evaluated |
38 (22.9) |
HCC staging at time T0, No (%) |
|
Child-Pugh Score |
|
A |
138 (83.1) |
B |
25 (15.1) |
C |
3 (1.8) |
MELD |
9.7 (3.47) |
BCLC, No (%) |
|
A |
65 (39.2) |
B |
30 (18.1) |
C |
64 (38.6) |
D |
7 (4.2) |
HCC treatments |
|
HCC main treatment/therapy, No (%) |
|
Curative |
94 (56.6) |
Palliative |
58 (34.9) |
Other/unknown |
14 (8.4) |
Outcome variable |
|
Recurrence, No (%) |
|
Absent |
57 (34.3) |
Present |
109 (65.7) |
Quantitative variables were expressed as mean (SD), qualitative variables as number (%) unless otherwise specified.
Table 1: Demographic and clinical characteristics of the patients.
3.2 Selection of the Most Suitable Model Building
In general, in the ML models, it is possible to define a specific function to choose the optimal tuning parameters. By default, the algorithm chooses the tuning parameter associated with the “best” performance (in our case the largest). Moreover, it is possible to use the "one standard error" rule of Breiman et al. [4], who suggest that the tuning parameter associated with the best performance may overfit. They advise that the simplest model within one standard error of the empirically optimal model is the better choice. All the models adopted in this study have been trained both with the “best” rule and with the “one standard error” rule explained above.
The k-NN is an algorithm used in pattern recognition for the classification of objects based on the characteristics of the objects close to the one considered. It is the simplest algorithm among those used in machine learning [36]. In our study, the model selected with the “best” rule and with the “one’” rule coincide and the tuning parameter selected is equal to 1. If the tuning parameter k is equal to 1 then the object is assigned to the class of its neighbour. In this specific case, it is called "the nearest neighbour algorithm". Table 2 shows that the k-NN is inaccurate for our data (0.5 < AUC ≤ 0.7). It was expectable as it is known from the literature that this model produces not accurate results. This model was considered as a benchmark for this study.
K-NN (oneSE = best) |
RF (best) |
RF (oneSE) |
SVM (best) |
SVM (oneSE) |
ENET (best) |
ENET (oneSE) |
|
Computational time (seconds) |
13.51 |
509.45 |
338.39 |
254.06 |
248.52 |
1,042.82 |
911.28 |
Tuning parameters |
k = 1 |
mtry = 11 |
mtry = 5 |
C = 3.8 |
C = 2.84 |
alpha = 0.942 |
alpha = 0.706 |
lambda = 0.013 |
lambda = 0.044 |
||||||
Balanced precision |
0.586 |
0.685 |
0.65 |
0.587 |
0.587 |
0.666 |
0.73 |
Balanced recall |
0.581 |
0.685 |
0.665 |
0.593 |
0.593 |
0.6 |
0.716 |
Balanced accuracy |
0.581 |
0.685 |
0.665 |
0.593 |
0.593 |
0.6 |
0.716 |
F1 balanced |
0.584 |
0.685 |
0.658 |
0.59 |
0.59 |
0.631 |
0.723 |
AUC value |
0.587 |
0.712 |
0.641 |
0.564 |
0.564 |
0.551 |
0.614 |
Table 2: Machine Learning models results
According to the Random Forest (RF) model trained with the “best” rule, there seems to be overfitting. The mtry equal to 11 seems too high, loosing quite a bit in terms of model accuracy. According to this, the model trained with the “one standard error” rule seems to be faster even if something is lost in terms of test set accuracy (Table 2). In general, the model trained with the “best” rule seems a moderately accurate model in the test set (0.7
3.3 Performance of the Recurrence P of the Most Suitable Model Building
According to the obtained results, the Random Forest model seems to be the most suitable for our data (AUC value: 0.712). The variable importance analysis was assessed using the average decrease in the nodes’ impurity measured by the Gini index during the construction of the random forest model [36]. Age at diagnosis, (Model for End-Stage Liver Disease) (MELD), anamnestic obesity, type of diagnosis, BMI, and BCLC were the six most important variables for recurrence prediction found in this analysis since they are associated with the highest Gini importance. To better understand the effects of the most important variables over classification results, the partial dependence plots [36] for the top 5-predictors have been explored (Figure 2). These plots give a graphical depiction of the marginal effect of a variable on the class probability. Greater y-values indicate that observation for a specific variable is associated with a higher probability for classifying new instances as recurrence. For age at diagnosis the Partial Dependence Plot (PDP) shows an increasing probability from about 50 to 70 years and then a decreasing probability. Conversely, lower MELD score is associated with a higher probability for a relapse (Figure 2). Moreover, surveillance or a casual diagnosis of the HCC is associated with a higher relapse probability compared to symptomatic diagnosis, as well as the absence of previous obesity (Figure 2). A low BMI is associated with a higher recurrence probability. Finally, a higher recurrence probability was also demonstrated for BCLC stage B patients. The learning curve is a plot that relates the accuracy of the learning system (ROC in our case) and the number of training examples used for learning [5]. The learning curve is useful for many purposes including comparing different algorithms, choosing model parameters during design, adjusting optimization to improve convergence, and determining the amount of data used for training. The learning curve (Figure 3) shows that as the training set increases, the quality of the prediction improves (ROC). Therefore, it may make sense to increase the number of cases to improve the performance of the model and make it more generalizable on new data.
4. Discussion
Artificial intelligence and machine learning have the potential to revolutionize the management of cancer patients by predicting their clinical course and outcome. In the past two years, AI methods have been published for the prediction of overall survival and treatment outcomes in HCC. To date, however, there are few clinical prognostic factors capable of predicting HCC recurrence. For this reason, we have performed this study with the aim to establish a new ML model for predicting outcome of HCC patients. ML models are capable to include a large amount of clinical, histological, radiological as well as molecular variables compared to traditional statistical models. The most important challenge in using ML is the careful selection and validation of algorithms to create a predictive model In this study, considering a large number of variables (socio-demographic, diagnosis of HCC, patient's medical history, comorbidities, residual liver function, HCC staging at diagnosis, treatments), including patients` outcome, we developed a supervised ML model to predict HCC recurrence. Moreover, six variables that can predict the recurrence of HCC, regardless of the type of treatment, have been identified. Among the three non-parametric models (Random Forest (RF), Support Vector Machine (SVM) and K-Nearest Neighbors (KNN)) and the parametric one (ENET) adopted, the Random Forest algorithm appears to be the most suitable for predicting the risk of HCC in our data. The six most important variables predicting HCC recurrence according to this model are age at diagnosis, MELD, the absence of previous obesity, type of diagnosis, BMI, and BCLC stage. According to our model instead, the type of treatment does not appear among the most predictive parameters. In our study, the likelihood of HCC recurrence increases from age 50 to 70 and then decreases. Cho E. et al. [40] described distinct clinical features among elderly HCC patients that could explain this finding. Elderly patients with HCC had fewer HCC nodules than younger patients and less liver fibrosis [40]. Furthermore, cancers in elderly patients tend to be more encapsulated, well-differentiated, and associated with less vascular invasion [40]. However, the size of HCC nodules in elderly patients was larger than that found in younger patients most likely due to the absence of regular HCC surveillance [40]. According to the model used, patients who did not have previous obesity and were underweight have a higher probability of HCC recurrence. Although this finding may be unexpected, 88% of the population in our study developed HCC on cirrhosis, and sarcopenia is a well-known characteristic of cirrhotic patients. Chang KV et al. [41] demonstrated that muscle loss is associated with increased all-cause mortality and tumor recurrence in HCC patients. Furthermore, we found that lower MELD values are associated with a higher likelihood of recurrence while both surveillance and random diagnosis of HCC are associated with a higher likelihood of recurrence. These results could be determined by the small sample size and the characteristics of our population. Survival in patients with chronic liver disease and successfully treated HCC is mainly affected by early hepatic decompensation (cit.). Longterm preservation of liver function may allow for the emergence of HCC and therefore of initiating a specific treatment (cit.). Finally, the learning curve shows that as the training set increases, accuracy improves. This could be promising for increasing sample size and obtaining more generalizable models in an independent data set. The present study had several limitations. The analysis of the results may have been limited by the sample size. Second, the few histological data may not emerge as a prognostic factor. In conclusion, AI and the models obtained with ML, analyzing a large number of variables, could provide predictive models that help predict the risk of recurrence. This study represents a "proof of concept" (also given the large number) and it opens the way to other studies with a larger number of patients, which also allows for differentiation by type of treatment.
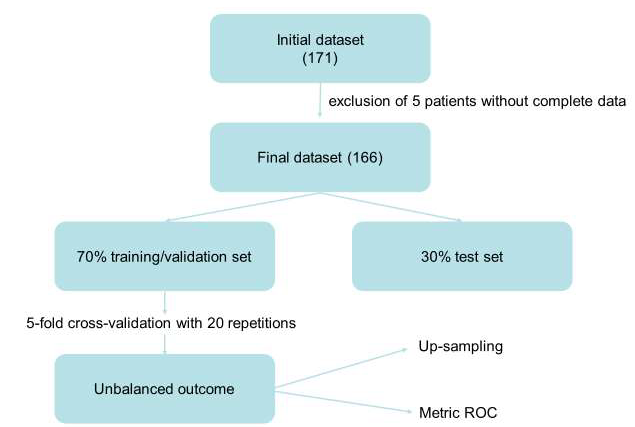
Figure 1: Model Building of Machine Learning
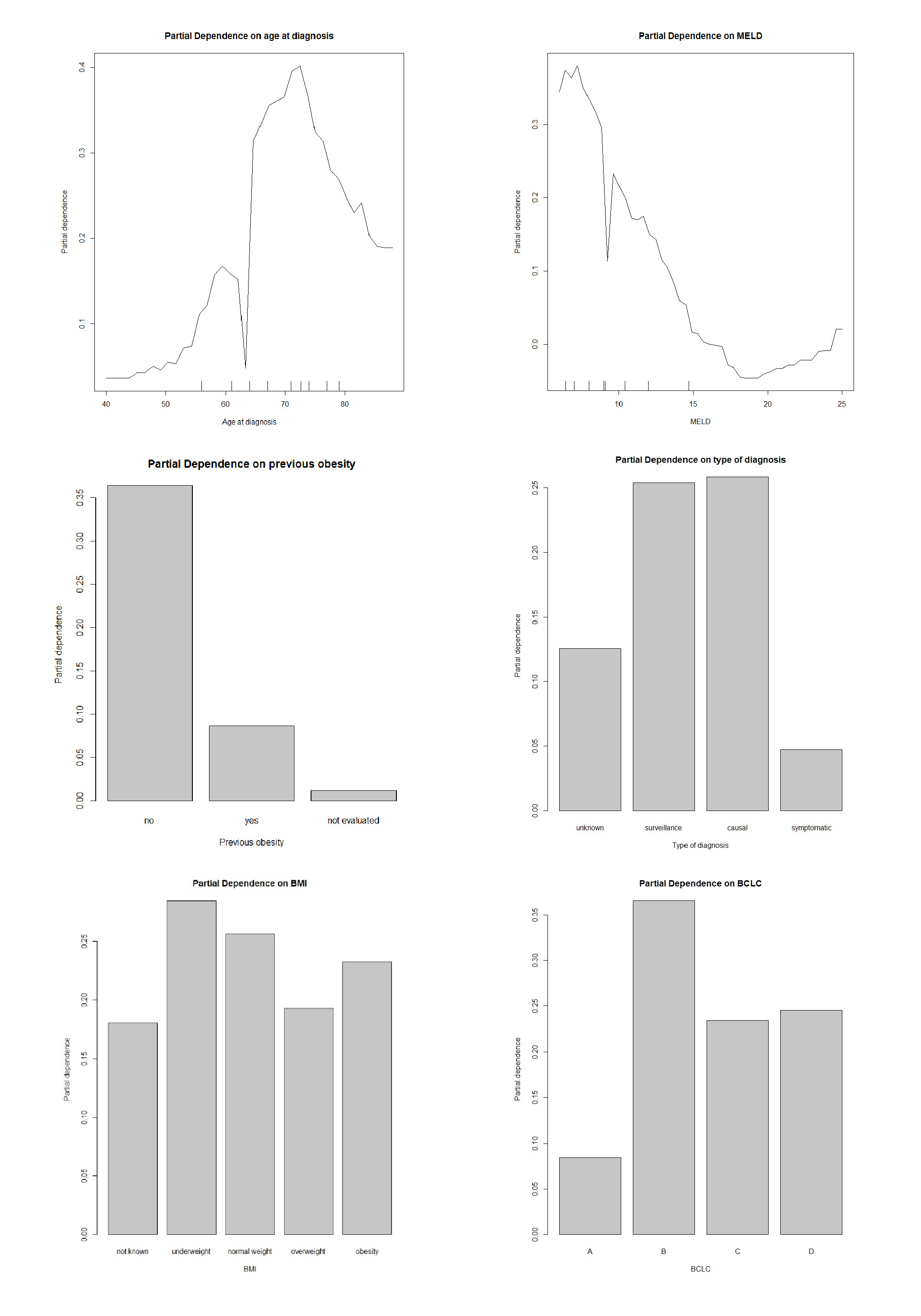
Figure 2: Partial dependence plots
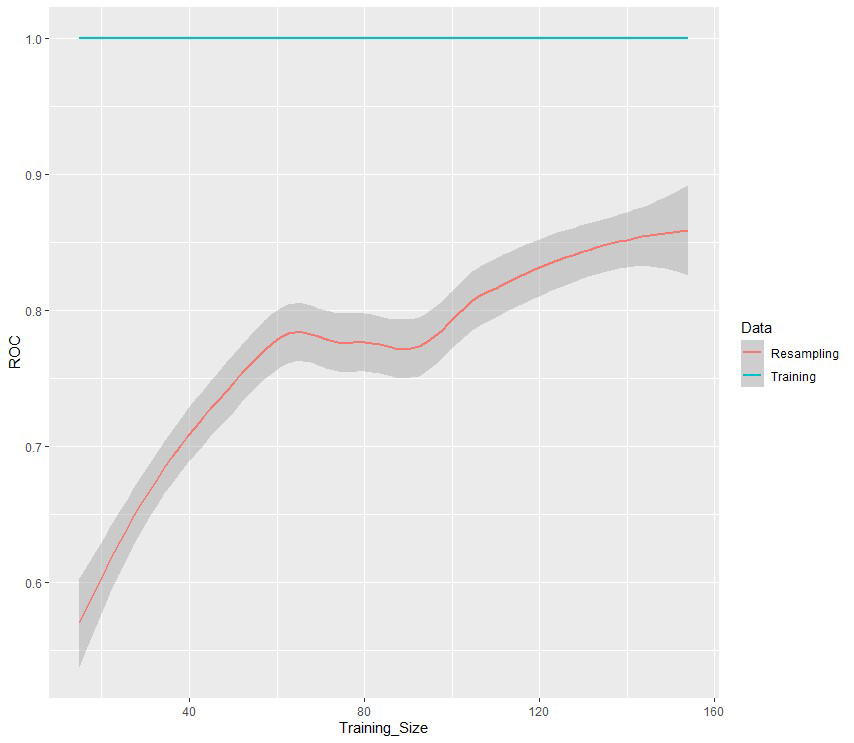
Figure 3: The learning curve of the Random Forest model
References
- International Agency for Research on Cancer. Globocan 2018. IARC (2020).
- Sagnelli E, Macera M, Russo A, et al. Epidemiological and etiological variations in hepatocellular carcinoma. Infection 48 (2020): 7-17.
- Llovet JM, Brú C, Bruix J. Prognosis of hepatocellular carcinoma: the BCLC staging classification. Semin Liver Dis 19 (1999): 329-338.
- Breiman, Friedman, Olshen, et al. (1984) Classification and Regression Trees. Wadsworth.
- Meek C, Thiesson B, Heckerman D. The Learning-Curve Sampling Method Applied to Model-Based Clustering. Journal of Machine Learning Research 2 (2002): 397.
- Vitale A, Lai Q, Farinati F, et al. Utility of Tumor Burden Score to Stratify Prognosis of Patients with Hepatocellular Cancer: Results of 4759 Cases from ITA.LI.CA Study Group. J Gastrointest Surg 22 (2018): 859-871.
- Lai Q, Vitale A, Halazun K, et al. Identification of an Upper Limit of Tumor Burden for Downstaging in Candidates with Hepatocellular Cancer Waiting for Liver Transplantation: A West-East Collaborative Effort. Cancers (Basel) 12 (2020): 452.
- European Association for the Study of the Liver. European Association for the Study of the Liver. EASL Clinical Practice Guidelines: Management of hepatocellular carcinoma. J Hepatol 69 (2018): 182-236.
- Amisha, Malik P, Pathania M, et al. Overview of artificial intelligence in medicine. J Family Med Prim Care 8 (2019): 2328-2331.
- Dong Y, Zhou L, Xia W, et al. Preoperative Prediction of Microvascular Invasion in Hepatocellular Carcinoma: Initial Application of a Radiomic Algorithm Based on Grayscale Ultrasound Images. Front Oncol 10 (2020): 353.
- Ma X, Wei J, Gu D, et al. Preoperative radiomics nomogram for microvascular invasion prediction in hepatocellular carcinoma using contrast-enhanced CT. Eur Radiol 29 (2019): 3595-3605.
- Xu X, Zhang HL, Liu QP, et al. Radiomic analysis of contrast-enhanced CT predicts microvascular invasion and outcome in hepatocellular carcinoma. J Hepatol 70 (2019): 1133-1144.
- Ji GW, Zhu FP, Xu Q, et al. Machine-learning analysis of contrast-enhanced CT radiomics predicts recurrence of hepatocellular carcinoma after resection: A multi-institutional study. EBioMedicine 50 (2019): 156-165.
- Zhou W, Zhang L, Wang K, et al. Malignancy characterization of hepatocellular carcinomas based on texture analysis of contrastenhanced MR images. J Magn Reson Imaging 45 (2017): 1476-1484.
- Liang JD, Ping XO, Tseng YJ, et al. Recurrence predictive models for patients with hepatocellular carcinoma after radiofrequency ablation using support vector machines with feature selection methods. Comput Methods Programs Biomed 117 (2014): 425-434.
- Morshid A, Elsayes KM, Khalaf AM, et al. A machine learning model to predict hepatocellular carcinoma response to transcatheter arterial chemoembolization. Radiol Artif Intell (2019): e180021.
- Peng J, Kang S, Ning Z, et al. Residual convolutional neural network for predicting response of transarterial chemoembolization in hepatocellular carcinoma from CT imaging. Eur Radiol 30 (2020): 413-424.
- Liu D, Liu F, Xie X, et al. Accurate prediction of responses to transarterial chemoembolization for patients with hepatocellular carcinoma by using artificial intelligence in contrastenhanced ultrasound. Eur Radiol 30 (2020): 2365-2376.
- Abajian A, Murali N, Savic LJ, et al. Predicting Treatment Response to Image-Guided Therapies Using Machine Learning: An Example for Trans-Arterial Treatment of Hepatocellular Carcinoma. J Vis Exp (2018): 58382.
- Ziv E, Yarmohammadi H, Boas FE, et al. Gene Signature Associated with Upregulation of the Wnt/β-Catenin Signaling Pathway Predicts Tumor Response to Transarterial Embolization. J Vasc Interv Radiol 28 (2017): 349-355.
- Mähringer-Kunz A, Wagner F, Hahn F, et al. Predicting survival after transarterial chemoembolization for hepatocellular carcinoma using a neural network: A Pilot Study. Liver Int 40 (2020): 694-703.
- Abajian A, Murali N, Savic LJ, et al. Predicting Treatment Response to Intra-arterial Therapies for Hepatocellular Carcinoma with the Use of Supervised Machine Learning- An Artificial Intelligence Concept. J Vasc Interv Radiol 29 (2018): 850-857.
- Morshid A, Elsayes KM, Khalaf AM, et al. A machine learning model to predict hepatocellular carcinoma response to transcatheter arterial chemoembolization. Radiol Artif Intell 1 (2019): e180021.
- Liu D, Liu F, Xie X, et al. Accurate prediction of responses to transarterial chemoembolization for patients with hepatocellular carcinoma by using artificial intelligence in contrastenhanced ultrasound. Eur Radiol 30 (2020): 2365-2376.
- Ibragimov B, Toesca D, Chang D, et al. Development of deep neural network for individualized hepatobiliary toxicity prediction after liver SBRT. Med Phys 45 (2018): 4763-4774.
- Wu CF, Wu YJ, Liang PC, et al. Disease-free survival assessment by artificial neural networks for hepatocellular carcinoma patients after radiofrequency ablation. J Formos Med Assoc 116 (2017): 765-773.
- Saillard C, Schmauch B, Laifa O, et al. Predicting Survival After Hepatocellular Carcinoma Resection Using Deep Learning on Histological Slides. Hepatology 72 (2020): 2000-2013.
- Zhou W, Chen H, Han W, et al. Prediction of hepatocellular carcinoma patient survival using machine learning classification rules. J Clin Oncol 37 (2019): e15649.
- Ji GW, Zhu FP, Xu Q, et al. Machine-learning analysis of contrast-enhanced CT radiomics predicts recurrence of hepatocellular carcinoma after resection: A multi-institutional study. EBioMedicine 50 (2019): 156-165.
- Wang W, Chen Q, Iwamoto Y, et al. Deep Learning- Based Radiomics Models for Early Recurrence Prediction of Hepatocellular Carcinoma with Multi-phase CT Images and Clinical Data. Annu Int Conf IEEE Eng Med Biol Soc 11 (2019): 4881-4884.
- Xu D, Sheng JQ, Hu PJ, et al. Predicting hepatocellular carcinoma recurrences: A data-driven multiclass classification method incorporating latent variables. J Biomed Inform 96 (2019): 103237.
- Feng ST, Jia Y, Liao B, et al. Preoperative prediction of microvascular invasion in hepatocellular cancer: a radiomics model using Gd-EOB-DTPA-enhanced MRI. Eur Radiol 29 (2019): 4648-4659.
- Tsilimigras DI, Mehta R, Moris D, et al. Utilizing Machine Learning for Pre- and Postoperative Assessment of Patients Undergoing Resection for BCLC-0, A and B Hepatocellular Carcinoma: Implications for Resection Beyond the BCLC Guidelines. Ann Surg Oncol 27 (2020): 866-874.
- Guo D, Gu D, Wang H, et al. Radiomics analysis enables recurrence prediction for hepatocellular carcinoma after liver transplantation. Eur J Radiol 117 (2019): 33-40.
- Branco P, Torgo L, Ribeiro RP. A Survey of Predictive Modelling under Imbalanced Distributions. ArXiv (2015).
- Hastie T, Tibshirani R, Friedman J. The Elements of Statistical Learning. Data Mining, Inference, and Prediction. Second edition, Springer Series in Statistics (2008).
- Max Kuhn. caret: Classification and Regression Training. R package version 6.0- 86 (2020).
- Habibzadeh F, Habibzadeh P, Yadollahie M. On determining the most appropriate test cut-off value: the case of tests with continuous results. Biochem Med (Zagreb) 26 (2016): 297-307.
- R Core Team. A language and environment for statistical computing. R Foundation for Statistical Computing, Vienna, Austria (2021).
- Cho E, Cho HA, Jun CH, et al. A Review of Hepatocellular Carcinoma in Elderly Patients Focused on Management and Outcomes. In Vivo (2019): 1411-1420.
- Chang KV, Chen JD, Wu WT, et al. Association between Loss of Skeletal Muscle Mass and Mortality and Tumor Recurrence in Hepatocellular Carcinoma: A Systematic Review and Meta-Analysis. Liver Cancer 7 (2018): 90- 103.
- Llovet JM, Zucman-Rossi J, Pikarsky E, et al. Hepatocellular carcinoma. Nat Rev Dis Primers 14 (2016): 16018.
- Rajula HSR, Verlato G, Manchia M, et al. Comparison of Conventional Statistical Methods with Machine Learning in Medicine: Diagnosis, Drug Development, and Treatment. Medicina (Kaunas) 56 (2020): 455.
- Huang S, Yang J, Fong S, et al. Artificial intelligence in cancer diagnosis and prognosis: Opportunities and challenges. Cancer Lett 471 (2020): 61-71.
- Cui S, Tseng HH, Pakela J, Ten Haken RK, El Naqa I. Introduction to machine and deep learning for medical physicists. Med Phys 47 (2020): e127-e147.