Short-Chain Fructooligosaccharides Improve Gut Microbiota Composition in Patients with Type 2 Diabetes. A randomized, Open-Label, Controlled Pilot Clinical Trial
Article Information
Gissel García1*, Duniesky Martínez2, Josanne Soto3, Lays Rodríguez4, Maricela Nuez5, Noraika Domínguez5, Emilio F. Buchaca4, Carlos Hernández2, Alina Sobrino2, Enrique R. Pérez2, Raúl J. Cano6*
1Pathology Department, Clinical Hospital " Hermanos Ameijeiras " (HHA). Calle San Lázaro No 701, Esq.a Belascoaín, Centro Habana, La Habana, P.C. 10400, Cuba
2Research and Development Department. Center for Genetic Engineering and Biotechnology of Sancti Spíritus (CIGBSS), Circunvalante Norte S/N, Olivos 3, Apartado Postal 83, Sancti Spíritus, P.C 60200, Cuba
3Clinical Laboratory Department, Clinical Hospital " Hermanos Ameijeiras " (HHA). Calle San Lázaro No 701, Esq.a Belascoaín, Centro Habana, La Habana, P.C. 10400, Cuba
4Internal Medicine Department, Clinical Hospital " Hermanos Ameijeiras " (HHA). Calle San Lázaro No 701, Esq.a Belascoaín, Centro Habana, La Habana, P.C. 10400, Cuba
5Endocrinology Department, Clinical Hospital " Hermanos Ameijeiras " (HHA). Calle San Lázaro No 701, Esq.a Belascoaín, Centro Habana, La Habana, P.C. 10400, Cuba
6Professor Emeritus, Biological Sciences Department, California Polytechnic State University, San Luis Obispo, CA 93407 USA
*Corresponding author: Gissel García, Pathology Department, Clinical Hospital " Hermanos Ameijeiras " (HHA). Calle San Lázaro No 701, Esq.a Belascoaín, Centro Habana, La Habana, P.C. 10400, Cuba.
Raúl J. Cano, Professor Emeritus, Biological Sciences Department, California Polytechnic State University, San Luis Obispo, CA 93407, USA.
Received: 15 May 2023; Accepted: 22 May 2023; Published: 08 June 2023
Citation: Gissel García, Duniesky Martínez, Josanne Soto, Lays Rodríguez, Maricela Nuez, Noraika Domínguez, Emilio F. Buchaca, Carlos Hernández, Alina Sobrino, Enrique R. Pérez, Raúl J. Cano. ShortChain Fructooligosaccharides Improve Gut Microbiota Composition in Patients with Type 2 Diabetes. A randomized, Open-Label, Controlled Pilot Clinical Trial. Journal of Biotechnology and Biomedicine. 6 (2023): 244-258.
View / Download Pdf Share at FacebookAbstract
In this study, the impact of KestoMix, a syrup containing short-chain fructooligosaccharides (scFOS) mainly 1-kestose, on clinical parameters and gut microbiota composition in type 2 diabetes (T2DM) patients was investigated. The study was conducted as a randomized, open label, controlled clinical trial involving 60 participants. The control group consumed microcrystalline cellulose capsules, while the KestoMix group consumed KestoMix (7.2 g) twice a day for 12 weeks. Stool and blood samples were collected from all the subjects. The gut microbial composition in feces was analyzed for 20 subjects by next-generation sequencing of the V3–V4 region of the bacterial 16S rRNA gene. KestoMix did not significantly modify the basal clinical parameters associated with T2DM, but it significantly reduced serum LDL-c concentration at week 12. In terms of gut microbial composition, the presence of Firmicutes was higher than Bacteroidetes in both groups, but KestoMix intake resulted in a reduction in the Firmicutes/Bacteroidetes ratio. Also, a significant increase (p=0.046) in the Bacteroidetes/Proteobacteria ratio compared to the control group was observed at day 84. Furthermore, KestoMix intake significantly stimulated the increase in Bifidobacterium, as well as Blautia and Lactobacillus (p=0.007, p=0.034 and p=0.016, respectively). While the alpha diversity of gut microbial composition was reduced after KestoMix intake, there was a change in the existing taxonomic proportions. Overall, this study highlights the positive impact of KestoMix on gut microbiota in T2DM patients. The bifidogenic effect of KestoMix may provide long-term benefits in complications associated with T2DM and other dysbiosis.
Keywords
Fructooligosaccharides; Prebiotic; Type 2 diabetes mellitus; Metagenomics sequencing; Gut microbiota
Fructooligosaccharides articles Fructooligosaccharides Research articles Fructooligosaccharides review articles Fructooligosaccharides PubMed articles Fructooligosaccharides PubMed Central articles Fructooligosaccharides 2023 articles Fructooligosaccharides 2024 articles Fructooligosaccharides Scopus articles Fructooligosaccharides impact factor journals Fructooligosaccharides Scopus journals Fructooligosaccharides PubMed journals Fructooligosaccharides medical journals Fructooligosaccharides free journals Fructooligosaccharides best journals Fructooligosaccharides top journals Fructooligosaccharides free medical journals Fructooligosaccharides famous journals Fructooligosaccharides Google Scholar indexed journals Prebiotic articles Prebiotic Research articles Prebiotic review articles Prebiotic PubMed articles Prebiotic PubMed Central articles Prebiotic 2023 articles Prebiotic 2024 articles Prebiotic Scopus articles Prebiotic impact factor journals Prebiotic Scopus journals Prebiotic PubMed journals Prebiotic medical journals Prebiotic free journals Prebiotic best journals Prebiotic top journals Prebiotic free medical journals Prebiotic famous journals Prebiotic Google Scholar indexed journals Type 2 diabetes mellitus articles Type 2 diabetes mellitus Research articles Type 2 diabetes mellitus review articles Type 2 diabetes mellitus PubMed articles Type 2 diabetes mellitus PubMed Central articles Type 2 diabetes mellitus 2023 articles Type 2 diabetes mellitus 2024 articles Type 2 diabetes mellitus Scopus articles Type 2 diabetes mellitus impact factor journals Type 2 diabetes mellitus Scopus journals Type 2 diabetes mellitus PubMed journals Type 2 diabetes mellitus medical journals Type 2 diabetes mellitus free journals Type 2 diabetes mellitus best journals Type 2 diabetes mellitus top journals Type 2 diabetes mellitus free medical journals Type 2 diabetes mellitus famous journals Type 2 diabetes mellitus Google Scholar indexed journals Metagenomics sequencing articles Metagenomics sequencing Research articles Metagenomics sequencing review articles Metagenomics sequencing PubMed articles Metagenomics sequencing PubMed Central articles Metagenomics sequencing 2023 articles Metagenomics sequencing 2024 articles Metagenomics sequencing Scopus articles Metagenomics sequencing impact factor journals Metagenomics sequencing Scopus journals Metagenomics sequencing PubMed journals Metagenomics sequencing medical journals Metagenomics sequencing free journals Metagenomics sequencing best journals Metagenomics sequencing top journals Metagenomics sequencing free medical journals Metagenomics sequencing famous journals Metagenomics sequencing Google Scholar indexed journals Gut microbiota articles Gut microbiota Research articles Gut microbiota review articles Gut microbiota PubMed articles Gut microbiota PubMed Central articles Gut microbiota 2023 articles Gut microbiota 2024 articles Gut microbiota Scopus articles Gut microbiota impact factor journals Gut microbiota Scopus journals Gut microbiota PubMed journals Gut microbiota medical journals Gut microbiota free journals Gut microbiota best journals Gut microbiota top journals Gut microbiota free medical journals Gut microbiota famous journals Gut microbiota Google Scholar indexed journals microbiota articles microbiota Research articles microbiota review articles microbiota PubMed articles microbiota PubMed Central articles microbiota 2023 articles microbiota 2024 articles microbiota Scopus articles microbiota impact factor journals microbiota Scopus journals microbiota PubMed journals microbiota medical journals microbiota free journals microbiota best journals microbiota top journals microbiota free medical journals microbiota famous journals microbiota Google Scholar indexed journals Faecalibacterium prausnitzii articles Faecalibacterium prausnitzii Research articles Faecalibacterium prausnitzii review articles Faecalibacterium prausnitzii PubMed articles Faecalibacterium prausnitzii PubMed Central articles Faecalibacterium prausnitzii 2023 articles Faecalibacterium prausnitzii 2024 articles Faecalibacterium prausnitzii Scopus articles Faecalibacterium prausnitzii impact factor journals Faecalibacterium prausnitzii Scopus journals Faecalibacterium prausnitzii PubMed journals Faecalibacterium prausnitzii medical journals Faecalibacterium prausnitzii free journals Faecalibacterium prausnitzii best journals Faecalibacterium prausnitzii top journals Faecalibacterium prausnitzii free medical journals Faecalibacterium prausnitzii famous journals Faecalibacterium prausnitzii Google Scholar indexed journals Epidemiological articles Epidemiological Research articles Epidemiological review articles Epidemiological PubMed articles Epidemiological PubMed Central articles Epidemiological 2023 articles Epidemiological 2024 articles Epidemiological Scopus articles Epidemiological impact factor journals Epidemiological Scopus journals Epidemiological PubMed journals Epidemiological medical journals Epidemiological free journals Epidemiological best journals Epidemiological top journals Epidemiological free medical journals Epidemiological famous journals Epidemiological Google Scholar indexed journals microbiome articles microbiome Research articles microbiome review articles microbiome PubMed articles microbiome PubMed Central articles microbiome 2023 articles microbiome 2024 articles microbiome Scopus articles microbiome impact factor journals microbiome Scopus journals microbiome PubMed journals microbiome medical journals microbiome free journals microbiome best journals microbiome top journals microbiome free medical journals microbiome famous journals microbiome Google Scholar indexed journals immunochemical articles immunochemical Research articles immunochemical review articles immunochemical PubMed articles immunochemical PubMed Central articles immunochemical 2023 articles immunochemical 2024 articles immunochemical Scopus articles immunochemical impact factor journals immunochemical Scopus journals immunochemical PubMed journals immunochemical medical journals immunochemical free journals immunochemical best journals immunochemical top journals immunochemical free medical journals immunochemical famous journals immunochemical Google Scholar indexed journals
Article Details
Introduction
The gut microbiota interacts with the host and regulates many metabolic processes on host physiology and metabolism, including glucose and lipid metabolism. Several reviews provided evidence of the relationship between the composition of the intestinal microbiota and the development of insulin resistance [1], type-2 diabetes mellitus [2] (Standards of Medical Care in Diabetes of American Diabetes Association (available at https://professional.diabetes.org/standards) and obesity[3]. Furthermore, the gut microbiota profiles of healthy persons differ from persons in metabolic disease states [4]. Because T2DM is a very common metabolic disease that can lead to various serious complications, and that affects the quality of life of a large population worldwide, new therapeutic approaches are needed to improve the incidence and severity of T2DM. Microbiota-targeted interventions to modulate gut microbiota and glucose metabolism are promising in the field of T2DM research [5]. Fructooligosaccharides (FOS) are considered prebiotics since they are substrates that are selectively utilized by host microorganisms, conferring a health benefit [6]. FOS could be synthesized from sucrose and the reaction product is a syrup with a mixture of carbohydrates including sucrose, glucose, fructose and scFOS [7]. Among the scFOS the best known are 1-kestotriose (GF2), 1,1-kestotetraose (GF3), and 1,1,1-kestopentaose (GF4), also named 1-kestose, nystose and fructosyl-nystose, respectively. The bifidogenic effect of scFOS is well known at well tolerated doses ranging from 2.5 to 10 g/d[8]. Besides Bifidobacterium, FOS consumption also increased the relative abundance of Operational Taxonomic Units belonging to Lactobacillus [9]. Enzymatically synthesized FOS from sugarcane syrup, supplemented into human fecal culture using a pH-controlled batch fermentation system, increased Bifidobacterium population, the steady Bacteroides/Prevotella growth and promoted an increase in the short-chain fatty acid production of the human intestinal microbiota [10]. Particularly 1-kestose, the smallest scFOS component, efficiently stimulates Faecalibacterium prausnitzii as well as bifidobacteria in humans [11]. A clinical study using 1-kestose suggests that may potentially ameliorate insulin resistance in overweight humans via the modulation of the gut microbiota [12]. The study of gut microbiota based on the traditional cultivation techniques only focus on a limited number of species [13]. However, with the development of next generation sequencing a better understanding of the interaction between gut microbiota and the metabolic processes of the host has been extensively studied, and it is well-established that dysbiosis can contribute to the development of metabolic disorders such as T2DM and obesity. To address this issue, microbiota-targeted interventions that modulate gut microbiota and glucose metabolism have been proposed as a potential therapeutic approach for T2DM.
The syrup FOS55 is a mix of carbohydrates manufactured by Florida, Camagüey, Cuba; registered under the name KestoMix that has not yet been used commercially in Cuba. The syrup is obtained by transfructosylation reaction from sucrose, with cane sugar as the raw material and a final composition of FOS around 55% (w/w) of total carbohydrates [7]. Taking in to account the reports of beneficial effects associated to FOS in promoting the growth of Bifidobacterium and Lactobacillus [14] as well as the increases in the production of short-chain fatty acids [15], we hypothesized that our product formulation could modify in a healthy manner the gut bacterial balance in T2DM patients. The primary aim of the study was to investigate if KestoMix improve or affect clinical parameters associated to T2DM like glycated hemoglobin A1c (HbA1c), fasting blood glucose (FBG), postprandial glucose – 2h (PPG), insulin; total cholesterol (Tc), triglycerides (TG), low-density lipoprotein cholesterol (LDL-c) and high-density lipoprotein cholesterol (HDL-c). The secondary aim was specifically to evaluate the effect of KestoMix, on the microbiome composition of patients diagnosed with T2DM.
Materials and Methods
Study design
The present randomized, open label controlled clinical trial study was conducted in patients with T2DM. The study was designed to investigate the effect of KestoMix prebiotic formulation on the gut microbiome composition and in clinical parameters associated with T2DM. This trial was conducted following to the Good Clinical Practice protocols as stated in the World Medical Association Declaration of Helsinki [16], and all procedures involving human subjects were approved under the Hermanos Ameijeiras Ethic Committee for Clinical Investigation and The Hermanos Ameijeiras Scientific Council. The KestoMix prebiotic formulation was evaluated and approved for it use by the Ethical Committee at the National Institute of Nutrition of Cuba and The Cuba Ministry of Health.
Subjects
The study included men and women between the ages of 30 and 65 years who had been diagnosed with T2DM following the Standards of Medical Care in Diabetes of American Diabetes Association (available at https://professional.diabetes.org/standards) [2] and who attended the Endocrine and Internal Medicine Division at Hermanos Ameijeiras Hospital. Before participating in the study, all volunteers provided a signed, written informed consent. Exclusion criteria were kidney disease, onco-proliferative diseases, and pregnancy. A total of 60 eligible patients with T2DM were randomly allocated to either the KestoMix group (KMG) or the control group (CG), with 30 individuals per group. The assignment to treatment groups was randomized using the Epidemiological Analysis from Tabulated Data (EpiData 3.1) software (Figure 1).

Figure 1: Figure 1 CONSORT Flow Diagram (Moher, Hopewell et al. 2012). Objective 1: Investigate if KestoMix improve or affect clinical parameters associated to T2DM like glicated hemoglobin A1c (HbA1c), fasting blood glucose (FBG), postprandial glucose – 2h (PPG), insulin; total cholesterol (Tc), triglycerides (TG), low-density lipoprotein cholesterol (LDL-c) and high-density lipoprotein cholesterol (HDL-c). Objective 2: Evaluate the effect of KestoMix, on the microbiome composition of patients diagnosed with T2DM
Trial intervention
The KestoMix prebiotic was orally administered to the KMG group at a dose of 5 mL (7.2 g) twice a day, 12 hours apart, for 12 weeks. The CG group received a capsule twice a day, 12 hours apart, for 12 weeks. Each bottle of the KestoMix contained 300 g of syrup with a composition (w/w) of 1-kestose 48.8%, nystose 6.8%, glucose 22.0%, sucrose 20.2%, and fructose 2.1%. The control group received capsules containing 370 mg of inulin, microcrystalline cellulose, D-mannitol, and stearic acid. Each group was monitored independently every 28 days for sample collection, supplement delivery, and clinical evaluation. To obtain biological samples, patients were instructed to fast for 8 hours, and venous blood samples were collected at baseline (day 1), day 28, day 56, and at the end of week 12. FBG and PPG were measured in all samples. HbA1c, insulin, Tc, TG, LDL-c and HDL-c were measured at baseline and week 12 using standard clinical methods. Blood samples for HbA1c determination were collected in K3EDTA tubes. Fecal samples from ten patients in each cohort group were collected for microbiome characterization studies at baseline and at the end of week 12. The samples were collected using DNA/RNA Shield™ Fecal Collection Tubes and stored at room temperature until processed. The frequency and consistency of stools were also noted by the volunteers.
Clinical Determinations
The clinical chemistry determinations were made in a modular immunochemical autoanalyzer Cobas 6000, from Roche Diagnostic that meets the requirements stipulated in Directive 98/79/EC of the European Parliament and the Council of the European Union (EU) on in vitro diagnostic medical devices, following the Standard Operating Procedures (SOPs) in force and with the quality control standards required. The range of quality control standard were FBG 4.2 – 7.0 mmol/L; PPG ≤ 10 mmol/L; Tc 3.6 – 5.2 mmol/L; TG 0.5 – 1.85 mmol/L; HDL-c ≥ 0.9 mmol/L; LDL-c 2.6 – 3.35 mmol/L; HbA1c ≤ 6%; Insulin 2.6 – 24.94 mIU/mL. Homeostatic Model Assessment of Insulin Resistance (HOMA-IR) was calculated with fasting plasma insulin (μU/mL) × fasting plasma glucose (mmol/L)/22.5.
Lipopolysaccharide (LPS) Determination
The determinations of serum LPS levels (results expressed in Endotoxin Units) of participating patients were made using the commercial kit ToxinSensorTM Endotoxin Detection System (Version 11242021), following the manufacturer's instructions (www.genscript.com).
Microbiome Analysis
16S amplicon sequencing
Targeted amplicon sequencing of the 16S rRNA V3-V4 regions within the ribosomal transcript was performed by EzBiome (Gaithersburg, MD, USA) using EzBiome’s protocols and workflow and Standard Operating Procedures.
Amplicon Taxonomic Assignment and Functional Prediction
Taxonomic profiling of 16S sequencing data was carried out by directly uploading forward and reverse paired end reads to the EzBioCloud microbiome taxonomy profiling platform (www.ezbiocloud.net) as described elsewhere [18]. Briefly, the cloud application of the EzBioCloud detects and filter out sequences of low quality regarding read length (<80 bp or >2,000 bp) and averaged Q values less than 25. Denoising and extraction of non-redundant reads are carried out using DUDE-Seq software [19]. The UCHIME [20] algorithm was applied against the EzBioCloud 16S chimera-free database to check and remove chimera sequencing. Taxonomic assignment was performed using the USEARCH program to detect and calculate the sequence similarities of the query single-end reads against the EzBioCloud 16S database. Sequencing reads are clustered into operational taxonomic units (OTUs) at 97% sequence similarity using the UPARSE algorithm [21]. Reads from each sample were clustered into many OTUs using the UCLUST [22] tool with the above-noted cutoff values. For the EzBioCloud 16S-based microbiome taxonomic profile (MTP) pipeline, the PICRUSt2 algorithm [23] was used to estimate the functional profiles of the microbiome identified using 16S rRNA sequencing. The raw sequencing reads were computed using the EzBioCloud 16S microbiome pipeline with default parameters and discriminating reads that were encountered in the reference database. The functional abundance profiles of the microbiome were annotated based on bioinformatics analyses, specifically by multiplying the vector of gene counts for each OTU by the abundance of that OTU in each sample, using the KEGG (Kyoto Encyclopedia of Genes and Genomes) [24] orthology and pathway database.
Amplicon Comparative Statistical and Bioinformatic Analyses
The study used EzBioCloud Application to perform subsampling, generation of taxonomy plots/tables, and rarefaction curves, as well as to calculate species richness, coverage, and alpha and beta diversity indices. The microbial richness was measured by abundance-based coverage estimators, Chao1, Jackknife, and the number of OTUs found in the MTP index. The Shannon, Simpson, and Phylogenetic α-diversity indices were applied to estimate the diversity for each group using the Wilcoxon rank-sum test [25]. Beta diversity was calculated with Bray-Curtis, UniFrac, and Generalized UniFrac distances based on the taxonomic abundance profiles [26]. Permutational multivariate analysis of variance (PERMANOVA) was applied to measure the statistical significance of β-diversity (Anderson 2014). Different groups were clustered with principal component analysis (PCoA) based on the Jaccard abundance distance metric. Kruskal-Wallis H test [27] and Effect Size (LEfSe) [28] analysis were performed to determine enrichment in the assigned taxonomic and functional profiles between groups. Taxonomic levels with LEfSe values higher than 2 at a p-value < 0.05 were considered statistically significant. The statistical analyses were conducted in R program (version 3.6.3 R Foundation for Statistical Computing, Vienna, Austria) using several packages, including 'vegan' v2.5-6, 'phyloseq', 'ggpubr', and 'ggplot2' v3.3.2. Rarefaction depth was set at 25,000 reads for microbiome analysis. All calculated p-values were two-tailed and considered statistically significant at p < 0.05. The Benjamini and Hochberg false discovery rate correction factor [29] was used to correct for errors in null hypothesis testing when conducting multiple comparisons.
Statistical analysis
All the values of anthropometric and clinical parameters were initially examined to determine whether they had a normal distribution using a Shapiro-Wilk test [30]. Non-normally distributed variables were log-transformed before analyses and outliers values were eliminated after Boxplot analyses. Treatment effects of KestoMix were assessed by comparing the value at baseline and day 84 for each subject using Paired-Samples t-test. Unpaired t-tests were performed for comparison between treatment with KestoMix and CG. For FBG and PPG, which were also measured at day 1, 28, 56 and 84, the comparisons between intra-subject factor (time) and between inter-subject factor (group) were analyzed with the application of a two-way Repeated Measure ANOVA. The correlations among the more abundant phyla and clinical data were performed using the Spearman’s test. P values less than 0.05 were considered statistically significant. All hypothesis tests were evaluated with a level of significance 0.05 with the Statistical Package for Social Sciences (SPSS) version 23.0.
Results
Study Population
A total of 60 T2DM patients were initially enrolled, with 30 assigned to the KestoMix group and 30 assigned to the control group (CG). However, five patients voluntarily withdrew from the study after the first visit due to personal or work-related reasons. The final sample size consisted of 28 patients in the KestoMix group and 27 patients in the CG (Figure 1). Baseline characteristics of the two groups are summarized in Table 1, and no significant differences were found between the groups for most variables, except for triglyceride levels, which were slightly higher in the CG and statistically significant compared to the KestoMix group (p=0.02).
Effect of KestoMix on anthropometric and clinical parameters
After 12-week intervention, while the CG significantly lost weight and BMI, KestoMix had no significant impact on body weight and BMI. Similarly, the changes of FBG, HbA1c, HOMA-IR and insulin concentrations over the course of the experimental period were not different between KMG and CG or day 1 and day 84 of the same group. Also, KestoMix had no effect on plasma glucose concentrations during 120 min in the PPG determination and showed no difference with the CG (Table 1).
Table 1: Anthropometric and physiological data for the participants at baseline and the end of the KestoMix intervention.
CG |
KMG |
|||||||
Day 1 |
Day 84 |
Day 1 |
Day 84 |
p1 |
p2 |
p3 |
p4 |
|
n = 27 |
n = 28 |
- |
||||||
T2DM Medication |
||||||||
M |
10 |
12 |
||||||
Insulin |
2 |
7 |
||||||
M + Insulin |
7 |
1 |
||||||
G + I/M |
6 |
3 |
||||||
No Medication |
2 |
5 |
||||||
Metric |
||||||||
Sex (m/f) |
13/14 |
14/14 |
- |
- |
- |
- |
||
Age (years) |
56.4 (54.1-58.7) |
58.0 (56.0-59.9) |
0.29 |
- |
- |
- |
||
High (m) |
1.66 (1.62-1.70) |
1.67 (1.63-1.71) |
0.89 |
- |
- |
- |
||
Weight (kg) |
85.1 (79.1-92.7) |
83.0 (77.3-89.1) |
83.0 (77.0-89.5) |
82.9 (77.3-89.0) |
0.55 |
0.98 |
0.00 |
0.93 |
BMI |
30.8 (28.7-33.1) |
29.8 (28.0-31.8) |
29.7 (27.7-31.9) |
29.6 (27.6-31.9) |
0.45 |
0.89 |
0.00 |
0.91 |
FBG |
7.4 (6.6-8.3) |
7.9 (7.2-8.7) |
7.0 (6.0-8.1) |
7.2 (6.4-8.0) |
0.6 |
0.08 |
0.12 |
0.71 |
PPG |
10.6 (9.3-12.1) |
11.1 (9.5-13.0) |
10.7 (8.7-13.1) |
10.1 (8.8-12.4) |
0.92 |
0.45 |
0.48 |
0.4 |
HbA1c |
7.2 (6.6-7.8) |
7.1 (6.4-7.9) |
6.9 (6.2-7.8) |
7.1 (6.4-7.9) |
0.74 |
0.44 |
0.92 |
0.67 |
Insulin |
16.4 (12.0-22.6) |
18.0 (14.3-22.5) |
19.2 (14.0-26.4) |
15.8 (11.8-21.3) |
0.39 |
0.79 |
0.33 |
0.19 |
HOMA-IR |
4.2 (2.9-6.1) |
5.3 (3.9-7.0) |
3.7 (2.7-5.2) |
3.6 (2.6-5.0) |
0.54 |
0.91 |
0.09 |
0.72 |
LPS |
0.42 (0.29-0.54) |
0.30 (0.21-0.40) |
0.36 (0.30-0.41) |
0.39 (0.33-0.46) |
0.29 |
0.10 |
0.02 |
0.33 |
Tc |
4.4 (4.1-4.6) |
5.1 (4.7-5.4) |
4.8 (4.4-5.2) |
4.7 (4.3-5.2) |
0.09 |
0.25 |
0.00 |
0.76 |
LDL-c |
2.7 (2.5-3.0) |
3.4 (3.1-3.8) |
3.2 (2.8-3.7) |
2.6 (2.2-3.0) |
0.10 |
0.00 |
0.00 |
0.00 |
HDL-c |
1.1 (1.0-1.2) |
1.1 (1.0-1.2) |
1.1 (1.0-1.2) |
1.0 (0.9-1.1) |
0.56 |
0.53 |
0.21 |
0.12 |
TG |
1.9 (1.6-2.2) |
1.1 (1.0-1.3) |
1.5 (1.2-1.9) |
1.3 (1.0-1.7) |
0.04 |
0.42 |
0.00 |
0.31 |
Values represent Mean (95% confidence interval); M, Metformin; M + Insulin, combination of Metformin and Insulin; G + I/M, combinations of Glibencamine and Metformin or Insulin. (m/f) male/female; BMI, body mass index (kg/m2); HbA1c (kg/m2); FBG (mmol/L); PPG (mmol/L); Insulin (μU/mL); LPS (EU/mL); Tc (mmol/L); LDL-c (mmol/L); HDL-c (mmol/L); TG (mmol/L). Values are mean ± SD. p1 Unpaired t-tests comparison between KMG and CG Day 1; p2 Unpaired t-tests comparison between KMG and CG day 84; p3 Paired t-tests comparison between day 1 and 84 of CG and p4 Paired t-tests comparison between day 1 and 84 of KMG; Significance difference p≤0.05.
FBG and PPG were also measured at day 1, 28, 56 and 84 (Figure 2) and they were analyzed with the two-way Repeated Measure ANOVA. The Mauchly's test of Sphericity was not significant neither for FBG (p=0.142) nor PPG (p=0.481). The comparison between the intra-subject factor (time) with sphericity assumed showed no difference for FBG (p=0.081) or PPG (p=0.937). The Box's M test for inter-group comparison showed homogeneity of covariance matrices, p=0.079 and p=0.172 for FBG and PPG, respectively. Then, comparison between CG and KMG showed not significant differences for FBG (p=0.338) nor PPG (p=0.764).
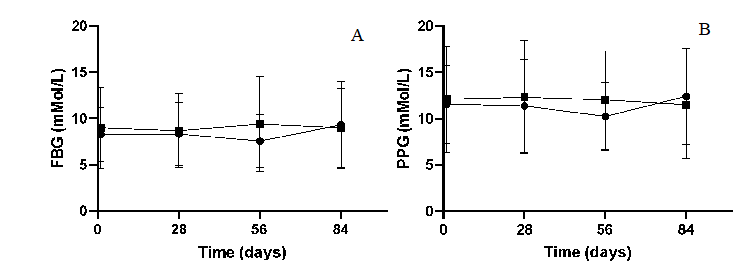
Figure 2: Variation of FBG (A) and PPG (B) in type 2 diabetic patients during 84 days of trial. Values represent mean ± standard deviation of CG (?) and KMG (?).The comparison between the intra-subject factor (time) showed no difference for FBG (p=0.081) nor PPG (p=0.937). The comparison between CG and KMG showed not significant differences for FBG (p=0.338) nor PPG (p=0.764). Two-way Repeated Measure ANOVA.
Supplementation of KestoMix has a different effect on lipid profile. No influence was observed on HDL-c, both in the CG and KMG. While Tc (4.4 mmol/L (4.1-4.6) vs 5.1 mmol/L (4.7-5.4) p < 0.05) and LDL-c (2.7 mmol/L (2.5-3.0) vs 3.4 mmol/L (3.1-3.8) p < 0.05) concentrations increased significantly in the CG, KestoMix significantly reduced serum LDL-c concentration at week 12 compared to that in the CG (2.6 mmol/L (2.2-3.0) vs. 3.4 mmol/L (3.1-3.8), p < 0.05) and the total amounts of cholesterol were unchanged in the KMG (p=0.76) (Table 1).
Analysis of the gut microbiota composition
To evaluate the effect of KestoMix on 20 T2DM patients gut microbiota, we analyzed the 16S ribosomal ARN sequences in stool samples collected at baseline (Day 1) and the end of the intervention (Day 84) from KMG and CG. The analysis of taxonomic profiles at the beginning and end of the study indicates a prevalence of microorganisms belonging to Bacteroidetes, Firmicutes, Proteobacteria, and Actinobacteria phyla, with Bacteroidetes and Firmicutes dominating over 90% of the total relative abundance, both in CG and KMG. The relative abundance of Actinobacteria increased from 3.2 to 6.1% of OTUs in the KMG, but decreased from 3.7 to 2.6% of OTUs, in the CG. While the relative abundance of Proteobacteria decreased in both groups, as well as the relative abundance of Firmicutes in the KMG, Bacteroidetes increased in both groups. Consequently, the Firmicutes/Bacteroidetes ratio decreased but Bacteroidetes/Proteobacteria ratio was significantly higher in the KMG in comparison to these of CG, at day 84 (p=0.046) (Figure 3A).
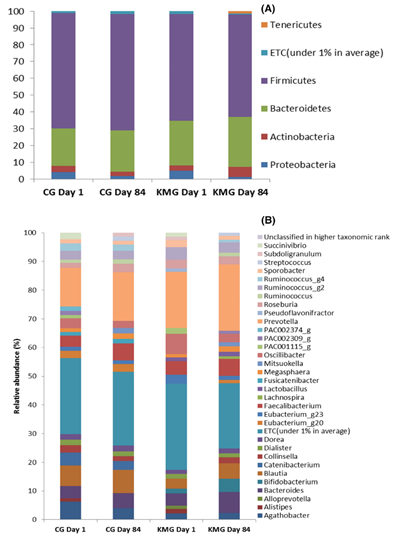
Figure 3: The relative abundance of gut bacteria found within metagenome datasets by phenotype. The relative abundance of major phyla (A) and genera (B).
Taxonomic analysis at the genus level revealed a high level of complexity, with as many as 20 genera comprising up to 70% of the gut microbiota, as shows Figure 3B. Prevotella, Blautia, Faecalibacterium, and Bacteroides are among the most abundant and important genera of indigenous bacteria that have established a symbiotic relationship with the human body over time. Following KestoMix treatment, there was a notable increase in the abundance of probiotic bacteria, such as Bifidobacterium and Lactobacillus. The MTP set analysis demonstrated a significant increase in the abundance of Blautia (p=0.034) and Lactobacillus (p=0.016) relative to day 1 following the KestoMix intervention. In addition, the KestoMix group exhibited a significant increase in Bifidobacterium abundance compared to day 1 (p=0.007) and the control group at day 84 (p=0.002). At the end of the study, no significant differences were observed in the abundance of Bacteroides and Prevotella compared to day 1, although their average relative abundance increased. The changes in the abundance of these genera were less pronounced in the control group, as depicted in Figure 4.
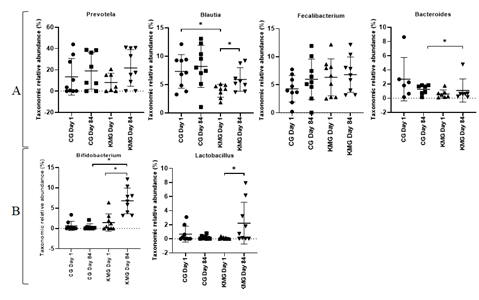
Figure 4: Bacterial species represented in high Relative Abundance in the gut microbiome at day 1 and 84 in the CG and KMG. (A) Indigenous bacteria (B) Probiotic bacteria. The relative abundance of genus was expressed as the media with the standard deviation. Unpaired t-tests comparison was used to analyze differences between KMG and CG at the same day; Paired t-tests comparison was used to analyze differences between day 1 and 84 of the same group. *(p-value < 0.05).
To evaluate the alpha-diversity between CG and KMG microbiome datasets, we employed three indices; Shannon (richness and evenness), Simpson (a diversity measure that considers both richness and evenness), and Chao1 (estimates the total richness). While Shannon and Simpson indices showed no differences between groups and days; Chao1 showed a significant difference between the KMG at day 84 with respect to KMG at day 1 (p=0.02) and with respect to CG at day 1 (p=0.00) and 84 (p=0.00), with the KMG at day 84 showing lower species richness in agreement with the lower OTUs detected in KMG after 84 days of intervention. Good's coverage, which reflects the depth of sequencing, was significantly lower in KMG compared to that in the CG at the beginning (p=0.00) and end (p=0.00) of the trial but increased significantly in the KMG at the end of treatment (p=0.01) (Figure 5). There were no statistically significant changes observed in Beta Diversity among the groups that were evaluated. UPGMA clustering demonstrated that the microbiome samples from individuals clustered together regardless of the date of sample collection. While the Unifrac distances between Day 1 and Day 84 in the KestoMix cohort differed, the difference was not statistically significant.
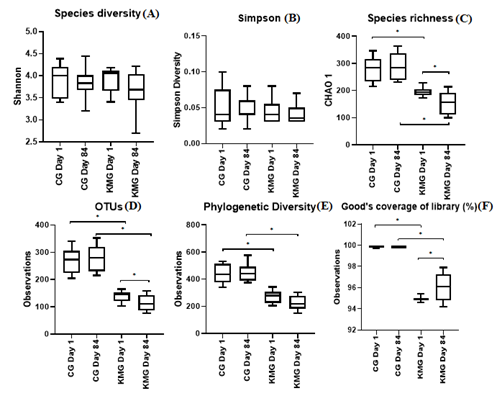
Figure 5: Alpha-diversity comparisons in the gut microbiomes. Boxplots for comparison of species diversity by Shannon index (A), species richness and evenness by Simpson index (B) and species richness by Chao1 index (C). Number of observed OTUs (D); Phylogenetic diversity (E) and Good's coverage of library (F). Unpaired t-tests comparison was used to analyze differences between KMG and CG at the same day; Paired t-tests comparison was used to analyze differences between day 1 and 84 of the same group. Boxplots indicate means and error bars denote maximum and minimum. *(p-value < 0.05).
To explore the correlations between the composition of the intestinal microbiota and clinical data, we analyzed the associations between more abundant phyla and clinical outcomes in both patient groups. A negative significant correlation was observed between the relative abundance of Actinobacteria and Tc (p=0.020, ρ=-0.387), as well as LDL-c (p=0.015, ρ=-0.404). Tc was also negatively correlated with the relative abundance of Proteobacteria (p=0.020, ρ=-0.387). Conversely, PPG was positively correlated with the relative abundance of Firmicutes (p=0.01, ρ=0.426), but negatively correlated with the relative abundance of Proteobacteria (p=0.034, ρ=-0.353), as shown in Table 2.
Actinobacteria |
Bacteroidetes |
Firmicutes |
Proteobacteria |
|
FBG |
-0.034 |
-0.185 |
-0.06 |
0.094 |
PPG |
-0.259 |
0.222 |
0.426** |
-0.353* |
Tc |
-0.387* |
0.205 |
0.21 |
-0.387* |
HDL-c |
0.058 |
-0.148 |
-0.041 |
0.069 |
LDL-c |
-0.404* |
0.234 |
0.145 |
-0.327 |
TG |
0.048 |
-0.169 |
-0.118 |
-0.058 |
HbA1c |
-0.058 |
-0.052 |
0.086 |
-0.12 |
Insulin |
-0.258 |
-0.026 |
0.223 |
0.01 |
HOMA-IR |
-0.205 |
-0.06 |
0.211 |
-0.014 |
LPS |
-0.102 |
-0.117 |
0.015 |
0.161 |
Weight |
0.161 |
0.163 |
-0.167 |
0.15 |
BMI |
0.226 |
0.053 |
-0.204 |
0.307 |
Data were analyzed by Spearman bivariate correlations. *p < 0.05, **p < 0.01. |
Table 2: Correlations between more abundant phyla and clinical characteristics.
Relative abundance of the KEGG orthologies (KOs)
Functional biomarkers were identified as putative using PICRUSt2 to infer relative abundances of functional genes from taxonomic data, and LEfSe was employed to identify discriminatory features that explain the functional and taxonomic differences between Day 1 and Day 84 in the KestoMix cohort (Table 3).
Relative Abundance of Genes |
|||||
Ortholog |
Definition |
p-value |
KestoMix Day1 |
KestoMix Day 84 |
Difference (%) |
K07492 |
putative transposase |
0.034 |
0.015 |
0.026 |
80.6 |
K21449 |
trimeric autotransporter adhesion |
0.011 |
0.065 |
0.097 |
50.6 |
K01854 |
UDP-galactopyranose mutase |
0.027 |
0.061 |
0.073 |
38.7 |
K03646 |
colicin import membrane protein |
0.011 |
0.035 |
0.046 |
33.0 |
K16786 |
energy-coupling factor transport system ATP-binding protein |
0.041 |
0.023 |
0.013 |
28.5 |
K02529 |
LacI family transcriptional regulator |
0.011 |
0.127 |
0.162 |
27.8 |
K06889 |
uncharacterized protein |
0.041 |
0.074 |
0.092 |
23.9 |
K08083 |
two-component system, LytTR family, response regulator AlgR |
0.027 |
0.038 |
0.046 |
22.5 |
K21064 |
5-amino-6-(5-phospho-D-ribityl amino)uracil phosphatase |
0.034 |
0.076 |
0.092 |
20.7 |
K03498 |
trk system potassium uptake protein |
0.027 |
0.060 |
0.072 |
19.7 |
K03657 |
DNA helicase II / ATP-dependent DNA helicase PcrA |
0.022 |
0.085 |
0.100 |
17.9 |
K02030 |
polar amino acid transport system substrate-binding protein |
0.018 |
0.116 |
0.134 |
15.5 |
K02003 |
putative ABC transport system ATP-binding protein |
0.034 |
0.128 |
0.147 |
14.5 |
K00845 |
Glucokinase |
0.011 |
0.123 |
0.134 |
9.3 |
K03609 |
septum site-determining protein MinD |
0.022 |
0.046 |
0.059 |
-23.8 |
K00529 |
3-phenylpropionate/trans-cinnamate dioxygenase ferredoxin reductase component |
0.034 |
0.033 |
0.020 |
-39.5 |
K07676 |
two-component system, NarL family, sensor histidine kinase RcsD |
0.041 |
0.040 |
0.024 |
-39.6 |
K02063 |
thiamine transport system permease protein |
0.006 |
0.029 |
0.040 |
-45.0 |
Table 3: Principal Functional Biomarkers identified by PICRUSt and LEfSe Analysis to Treated in the KestoMix cohort.
The analysis revealed that the most abundant KO was K07492 (80.6%), which encodes for the putative transposase enzyme. The second most expressed gene was K21449 (50.6%), which encodes for the trimeric autotransporter adhesin. Another important KO gene that provides insight into the healthy microbiome restoration in these individuals was detected at 38.7% (K01854). The expression of K03646 at 33% is also crucial for maintaining intestinal health induced by the KestoMix formulation.
Discussion
Effect of KestoMix on anthropometric and clinical parameters
The present study investigated the effects of KestoMix supplementation on glucose and lipids metabolism in T2DM patients. The study participants maintained their diabetes under control, as evidenced by their mean baseline FBG concentration of 7.2 mmol/L, which showed no significant differences between Days 1 and 84, even after a 12-week KestoMix intake. This observation is noteworthy, considering that the KestoMix composition includes glucose at 22.0% (w/w) and sucrose at 20.2% (w/w). It is also important to note that the control group received only 370 mg inulin, which is much lower than the recommended daily intake of 10 g necessary to achieve a prebiotic effect. Various studies on the effects of FOS or inulin consumption by type 2 diabetic patients on plasma glucose have reported divergent results [31-33]. In agreement with our results, Luo et al. (1996) reported that daily intake of 15-20 g FOS did not significantly affect blood glucose in patients with T2DM [34], in other study in obese women, 16 g inulin-type fructans/d did not significantly modify HbA1c, fasting glycemia and HOMA index [35]. Conversely, adverse effect on glucose metabolism, demonstrated by OGTT, has been reported for short-term intake of high dose FOS intervention in healthy young population [14]. However, trial design, cohort composition, and dose of prebiotics can also impact on the results. The FOS used in our trial was produced via the transfructosylation reaction from sucrose, with sucrose as the raw material [7]. In other trials, FOS were from different sources and companies Raftilose P95; from ORAFTI, Tienen, (Belgium) [36]; ACTILIGHT from Béghin Meiji Industries, Neuilly sur Seine, (France)[34]; Neosugar® from Meiji Seika Co (Japan) [37] and QHT-Purity95% from Quantum Hi-Tech (China) [14]. The effects of KestoMix supplementation on lipids metabolism in patients with type 2 diabetes was also investigated in the present work. The study found no significant effect of KestoMix supplementation on Tc, HDL-c and TG, but it tended to lower LDL-c. Similarly, other studies with inulin-type FOS showed constant concentrations of serum lipids in fasting subjects [38]. The reduction of LDL-c could be associated to the negative correlation observed between Actinobacteria and LDL-c.
Phyla composition of gut microbiota
Gut microbiota at the beginning and end of the study showed the prevalence of four main phyla: Firmicutes, Bacteroidetes, Proteobacteria and Actinobacteria, with Gram-negative Bacteroidetes (less than 30%) and Gram-positive/negative Firmicutes (more than 60%) dominating over 90% of the total relative abundance, both in CG and KMG of T2DM patients. These four dominant phyla have been reported both in T2DM and healthy controls [39]. However, the differences of phyla abundance in the intestinal microbiota composition between humans with T2DM and non-diabetic persons is controversial. The abundance of Firmicutes, Bacteroidetes, Proteobacteria and Actinobacteria phyla in T2DM or Metabolic syndrome has been reported without significant difference from healthy controls , however Ahmad et al., 2020 [40], revealed an increased abundance of Firmicutes in the microbiomes of obese-T2DM individuals while the proportions of phylum Firmicutes were significantly reduced in the diabetic group compared to the control group according to Larsen 2010 [4]. The Firmicutes/Bacteroidetes ratio in this study is in agreement with the recent evidence found in T2DM patients, this proportion, however, was also found in non-diabetic controls [41]. Our results contrast with those found in obese, T2DM and patients with Metabolic syndrome where Bacteroidetes is the dominant phylum [42]; but this high proportion of Bacteroidetes was also reported in healthy persons [39].
Influence of KestoMix on gut microbiota proportions
T2DM, obesity, metabolic syndrome and other disorders has been associated with low bacterial diversity. On the other hand, it is expected that FOS consumption increase microbiome diversity, since inulin type FOS, like 1-kestose, are considered fiber [35], however some studies in mice and human showed no significant differences in Shannon diversity between the placebo and those groups supplemented with FOS. In our study was observed a reduction in Shannon diversity by FOS supplementation, this result is similar to those reported by Kato et al. [43]. It is important to note that over half of the patients in each group used metformin, which has been shown to decrease the Shannon index [44]. After KestoMix consumption, the predominant phyla found in the basal phase were observed but also was detected the presence of around 1 % Tenericutes OTUs. Overall, this observation suggests that KestoMix intake does not disrupt or alter the original composition of the gut microbiome in T2DM patients, but only leads to changes in existing taxonomic proportions. Notably, in both groups, the relative abundance of Proteobacteria decreased and that of Bacteroidetes increased, while the KMG exhibited a decrease in Firmicutes. After KestoMix treatment, an increase in Bacteroidetes levels was observed due to the increase in Bacteroides and Prevotella, along with improvement in Blautia and Lactobacillus levels, although there was no increase in the relative abundance of Firmicutes. The KMG showed a reduction in the Firmicutes/Bacteroidetes ratio and a significant increase in the Bacteroidetes/Proteobacteria ratio compared to the CG at day 84. Several studies have shown that Proteobacteria have a negative impact on human health [45]. The higher abundance of Phylum Proteobacteria in the T2DM and the obese microbiome when compared to healthy controls has been reported. Proteobacteria may represent an imbalance in the gut microbial community and be a potential marker of dysbiosis [46]. A recent study on the meta-analysis of microbial community dynamics, employed a network to study the interactions through microbial association between healthy and disease gut microbiomes showed that Proteobacteria interactions were significantly enriched in all disease networks [40].
The present study revealed that the intestinal Gram-negative bacteria Bacteroidetes/Proteobacteria ratio was significantly higher (p=0.046) in the KMG in comparison to those of CG, at day 84. Lipopolysaccharides (LPS) are the main compounds of cell walls in Gram-negative bacteria [47]. LPS is one of the potent virulence factors of Gram-negative bacterial species and it is known as potent stimulators of inflammation, which can exhibit endotoxemia [4]. Increased level of Gram-negative gut bacteria correlates with high circulating LPS and high insulin resistance in T2DM [48]. Of note, the activity of LPS of Bacteroidetes is less effective compared with that of other Gram-negative bacteria [49]. Recently, Blautia has been of particular interest since accumulating evidence has demonstrated a link between this genus and the alleviation of inflammatory and metabolic diseases, and its association with the production of health-promoting compounds such as short-chain fatty acids (SCFAs) or antimicrobial peptides against specific microorganisms [50]. B. wexlerae, was identified as a commensal bacterium that is inversely correlated with obesity and type 2 diabetes mellitus [51] and is also associated to the decrease of insulin resistance in obese individuals [40]. In this study, a significant increase in Blautia genus (p=0.034), was also observed after KestoMix treatment. The effect of FOS on the Blautia population has shown discrepant results, a significant increase in the relative abundance of Blautia in the FOS high-dose group was observed in mice. Otherwise, studies in human showed no significant difference in the absolute number of Blautia during FOS intake or a decrease compared to the placebo group after 1-kestose consumption [12]. The relative abundance of Actinobacteria increased in the KMG but decreased in the CG. The increased level of Actinobacteria following KestoMix treatment was due to the increase in Bifidobacterium mainly B. adolescentis and B. longum. Liu and co-workers also observed in healthy young population an increase at phylum level of Actinobacteria by a rise in the relative abundance of Bifidobacterium after FOS and GOS intake [14]. The bifidogenic activity of inulin-type FOS, mainly 1-kestose, have been extensively reported. The scFOS consumption of 10 g/d dose significantly increased fecal bifidobacteria in healthy volunteers consuming their usual diet [52]. The relative abundance of fecal Bifidobacterium was significantly increased in 1-kestose-supplemented obesity-prone participants compared to that in controls [12], 1-kestose levels showed substantial rises in Bifidobacterium and Faecalibacterium in the in vitro gut microbiota composition of normal-weight individuals [53]. These probiotic bacteria correlated well with carbohydrate metabolism as well as a positive impact on the production of SCFAs [15] and with gut microbiota recovery following antibiotic intake, specifically B. adolescentis [54]. In this study, it was observed that KestoMix intake resulted in an increase in populations of Bifidobacterium, as well as Bacteroides and Prevotella. These three bacteria are known to metabolize FOS to produce acetate, as previously reported [10], largely because most enteric bacteria including Bacteroides, Prevotella and Bifidobacterium produce acetate from pyruvate via the acetyl-CoA pathway [55]. The larger production of lactic acid and acetate by Bifidobacteria is obtained by a preferential fermentation of 1-kestose instead of nystose or FOS with higher degree of polymerization [11]. The release of acetate, a short-chain fatty acid, is known to inhibit the growth of certain pathogens such as Clostridium histolyticum, which are sensitive to low pH [56]. Additionally, acetate has been shown to reduce the adhesion of pathogens to the intestinal mucosa, which can help prevent infections [57]. However, a decrease in the number of beneficial bacteria such as Bifidobacterium can create a favorable environment for the colonization of harmful bacteria that release LPS. These LPS can induce the production of pro-inflammatory cytokines, which can weaken the intestinal wall and allow bacterial antigens to enter the bloodstream and organs. This can lead to subclinical inflammation and other health issues, such as insulin resistance and obesity [58]. The increased circulation of LPS in the body raises the concentration of triglycerides and glucose. The deficiency of Bifidobacterium contributes to obesity and insulin resistance in the progress of type 2 diabetes [59]. Therefore, even when no differences were observed in the LPS concentration in the KMG between day 1 and 84, the rise of Bacteroidetes/Proteobacteria ratio and the increased level of Actinobacteria likely due to the increase in Bifidobacterium could be beneficial in a long-term control LPS levels.
Relative abundance of the KEGG orthologues (KOs)
LEfSe analysis identified the ortholog gene K21449 in over 50% of the expression data analyzed. This gene codes for a trimeric autotransporter adhesin. This observation suggests that this gene can be used as a microbiome biomarker in healthy individuals who have high levels of Firmicutes, specifically the genera Blautia, Clostridium, Eubacterium, and Faecalibacterium [60]. Firmicutes is a phylum of bacteria commonly found in the human gut, and some members of these genera are known to have beneficial effects on human health. Overall, the identification of this ortholog gene and its association with certain bacterial genera may provide insight into the composition of the gut microbiome in healthy individuals, which could have implications for the development of strategies to promote gut health. Microbiome interrogation also identified an ortholog gene K01854, that was detected in 38.7% of the expression data analyzed. This gene is involved in the expression of UDP-galactopyranose mutase, an enzyme that plays a key role in galactose metabolism, as well as amino sugar and nucleotide sugar metabolism. This enzyme is important for the production of cellular energy and the modification of proteins and glycolipids. The expression of this gene is significantly higher in healthy controls compared to colorectal cancer patients. This finding suggests that the regulation of galactose metabolism and related metabolic pathways may play a role in maintaining a healthy gut microbiome. Overall, the identification of this ortholog gene and its association with healthy controls and colorectal cancer patients could have important implications for the development of new therapies and strategies to promote gut health and prevent colorectal cancer [60]. Both Bacteroides spp. and Bifidobacterium spp. play important roles in galactose metabolism in the gut, and they are known to have protective effects against inflammatory diseases [61, 62]. Bacteroides spp. are involved in the breakdown of complex polysaccharides, which can produce SCFAs that are beneficial for colon health [60]. Bifidobacterium spp. can metabolize galactose into SCFAs, which can provide energy for colonocytes and help to maintain the integrity of the gut barrier [63]. The expression of K03646 in 33% is also important for intestinal health induced by KestoMix formulation. This ortholog gene codified for the colicin import membrane protein with important antibacterial properties [64]. Colicin import membrane protein is a bacteriocin, a large and heterologous group of toxins with antibacterial properties but not considered as antibiotics. Among Gram-negative bacteria, the Enterobacteriaceae are the major producers of bacteriocins, which are classified in two categories: low molecular weight bacteriocins or microcins (less than 10 kDa), and high molecular weight bacteriocins including colicins produced by Escherichia coli (25 to 80 kDa) [65]. There is increasing interest in protein bacteriocin import; from a fundamental perspective to understand how folded proteins are imported into bacteria and from an applications perspective as species specific antibiotics to combat multidrug resistant bacteria.
Limitation of the study
This study has limitations, principally in the sample size of the population analyzed for microbiome composition. As indicated earlier, this study represents a pilot study from which to gather data for a larger study from which more in-depth information can be obtained regarding the microbiome of treated patients with KestoMix. This is particularly important in view that the diversity of human microbiota compositions is unique to each individual [66] and as such it is important to evaluate a larger sample size to ascertain the significant observations reported here. Another shortcoming and one that would be addressed in a larger, subsequent study is that the authors did not elaborate on the caloric intake and dietary pattens (proportion of plant vs animal-based diet) of participants as these may have an indirect effect in several biological parameters studied including cholesterol levels.
Conclusions
Based on the findings of the study, it appears that KestoMix is effective in promoting a favorable change in gut microbiome, specifically by increasing the populations of Bifidobacterium, Blautia, and Lactobacillus. These three genera have been classified as beneficial intestinal commensals, which suggest that the consumption of KestoMix could have positive effects on gut health. Although the study did not find significant effects on glucose and lipid metabolism in patients with T2DM, it is possible that a different formulation of KestoMix with lower sucrose or glucose concentrations could have different effects on these metabolic parameters. The study also suggests that the bifidogenic effect of KestoMix may confer long-term benefits in complications associated with T2DM and other dysbiosis. This is because the gut microbiota plays an important role in maintaining overall health and is known to be disrupted in individuals with T2DM and other metabolic disorders. By promoting the growth of beneficial bacterial populations, KestoMix may help to restore a healthy gut microbiota and reduce the risk of complications associated with dysbiosis. These observations will have to be validated in a subsequent study with a greater, statistically valid sample size of fecal samples earmarked for microbiome analysis.
Availability of data and materials
The raw sequencing data presented in this study are deposited in the NCBI Sequence Read Archive (SRA), (https://www.ncbi.nlm.nih.gov/) Search as KestoMix.
Competing interests
The corresponding author (RJC) was Chief Scientific Officer at The BioCollective, LLC, a firm that contributed funds the clinical study and remained a paid consultant for the duration of the study The BioCollective, LLC had no commercial interest in KestoMix, the test substance reported herein and no involvement in the design, development, and manufacturing of KestoMix. The remaining authors have no conflicts of interest to declare.
Funding
This project was funded, in part, by a donation of reagents and supplies by The BioCollective, LLC, Denver, CO. The Hermanos Ameijeiras Hospital, Havana, Cuba donated personnel time, resources, and project management as a contribution in kind.
Acknowledgements
We would like to express our gratitude to Dr. Miguel H. Estévez del Toro, Director of the Hermanos Ameijeiras Hospital and to Eng.Yurailis Reyes, for their support in initiating and conducting the study. We would also like to express our gratitude to The BioCollective management for contributing the necessary reagents for this study.
Data Availability Statement
All data relating to the contents of this report will be made readily available upon request to the Corresponding Author.
References
- Cani PD, Amar J, Iglesias MA, et al. Metabolic endotoxemia initiates obesity and insulin resistance. Diabetes 56 (2007): 1761-1772.
- Petersmann A, Müller-Wieland D, Müller UA, et al. Definition, Classification and Diagnosis of Diabetes Mellitus. Exp Clin Endocrinol Diabetes 127 (2019): S1-S7.
- Portune KJ, Benítez-Páez A, Del Pulgar EMG, et al. Gut microbiota, diet, and obesity-related disorders-The good, the bad, and the future challenges. Molecular nutrition & food research 61 (2017): 1600252.
- Larsen N, Vogensen FK, Van Den Berg FW, et al. Gut microbiota in human adults with type 2 diabetes differs from non-diabetic adults. J PloS one 5 (2010): e9085.
- Woldeamlak B, Yirdaw K, Biadgo B. Role of gut microbiota in type 2 diabetes mellitus and its complications: Novel insights and potential intervention strategies. The Korean Journal of Gastroenterology 74 (2019): 314-320.
- Gibson GR, Hutkins R, Sanders ME, et al. Expert consensus document: The International Scientific Association for Probiotics and Prebiotics (ISAPP) consensus statement on the definition and scope of prebiotics. Nature reviews Gastroenterology & hepatology 14 (2017): 491-502.
- Hernández L, Menéndez C, Pérez ER, et al. Fructooligosaccharides production by Schedonorus arundinaceus sucrose: sucrose 1-fructosyltransferase constitutively expressed to high levels in Pichia pastoris. Journal of biotechnology 266 (2018): 59-71.
- Bouhnik Y, Raskine L, Simoneau G, et al. The capacity of short-chain fructo-oligosaccharides to stimulate faecal bifidobacteria: a dose-response relationship study in healthy humans. Nutrition journal 5 (2006): 1-6.
- Tandon D, Haque MM, Gote M, et al. A prospective randomized, double-blind, placebo-controlled, dose-response relationship study to investigate efficacy of fructo-oligosaccharides (FOS) on human gut microflora. Scientific Reports 9 (2019): 5473.
- Hajar-Azhari S, Abd Rahim MH, Sarbini SR, et al. Enzymatically synthesised fructooligosaccharides from sugarcane syrup modulate the composition and short-chain fatty acid production of the human intestinal microbiota. Food Research International 149 (2021): 110677.
- Tochio T, Kadota Y, Tanaka T, et al. 1-Kestose, the smallest fructooligosaccharide component, which efficiently stimulates Faecalibacterium prausnitzii as well as Bifidobacteria in humans. J Foods 7 (2018): 140.
- Watanabe A, Tochio T, Kadota Y, et al. Supplementation of 1-Kestose Modulates the Gut Microbiota Composition to Ameliorate Glucose Metabolism in Obesity-Prone Hosts. Nutrients 13 (2021): 2983.
- Roberfroid M, Gibson GR, Hoyles L, et al. Prebiotic effects: metabolic and health benefits. British Journal of Nutrition 104 (2010): S1-S63.
- Liu F, Li P, Chen M, et al. Fructooligosaccharide (FOS) and Galactooligosaccharide (GOS) Increase Bifidobacterium but Reduce Butyrate Producing Bacteria with Adverse Glycemic Metabolism in healthy young population. Scientific Reports 7 (2017): 11789.
- O'Callaghan A, van Sinderen D. Bifidobacteria and Their Role as Members of the Human Gut Microbiota. Frontiers in Microbiology 7 (2016):925.
- Raj GM. Ethical Issues Related to Medical Research on Human Participants. In Lakshmanan M, Shewade DG, Raj GM (eds): Introduction to Basics of Pharmacology and Toxicology: Volume 3: Experimental Pharmacology: Research Methodology and Biostatistics. Singapore: Springer Nature Singapore 10 (2022): 819-837.
- Moher D, Hopewell S, Schulz KF, et al. CONSORT 2010 explanation and elaboration: updated guidelines for reporting parallel group randomised trials. Research Methods & Reporting 10 (2012): 28-55.
- Yoon S-H, Ha S-M, Kwon S, et al. Introducing EzBioCloud: a taxonomically united database of 16S rRNA gene sequences and whole-genome assemblies. International journal of systematic and evolutionary microbiology 67 (2017): 1613.
- Lee B, Moon T, Yoon S, et al. DUDE-Seq: Fast, flexible, and robust denoising for targeted amplicon sequencing. PLOS ONE 12 (2017): e0181463.
- Edgar RC, Haas BJ, Clemente JC, et al. UCHIME improves sensitivity and speed of chimera detection. Bioinformatics 27 (2011): 2194-2200.
- Edgar RC. UPARSE: highly accurate OTU sequences from microbial amplicon reads. Nature Methods 10 (2013): 996-998.
- Prasad DV, Madhusudanan S, Jaganathan SJAJoE, et al. uCLUST-a new algorithm for clustering unstructured data. In. J. of Advance Research, Ideas and Innovations in Technology 10 (2015): 2108-2117.
- Yin Y, Wang J. Predictive functional profiling of microbial communities in fermentative hydrogen production system using PICRUSt. International Journal of Hydrogen Energy 46 (2021): 3716-3725.
- Kanehisa M, Goto S. KEGG: Kyoto Encyclopedia of Genes and Genomes. Nucleic Acids Research 28 (2000): 27-30.
- Rosner B, Glynn RJ, Lee MLTJB. The Wilcoxon signed rank test for paired comparisons of clustered data. Biometrics 62 (2006): 185-192.
- Dominianni C, Wu J, Hayes RB, et al. Comparison of methods for fecal microbiome biospecimen collection. BMC Microbiology 14 (2014): 103.
- Daniel WW. Friedman two-way analysis of variance by ranks. Applied nonparametric statistics 10 (1990): 262-274.
- Segata N, Waldron L, Ballarini A, et al. Metagenomic microbial community profiling using unique clade-specific marker genes. Nature Methods 9 (2012): 811-814.
- Robertson DS, Wildenhain J, Javanmard A, et al. onlineFDR: an R package to control the false discovery rate for growing data repositories. Bioinformatics 35 (2019): 4196-4199.
- Franzosa EA, McIver LJ, Rahnavard G, et al. Species-level functional profiling of metagenomes and metatranscriptomes. Nature Methods 15 (2018): 962-968.
- .Livesey G, Taylor R, Livesey HF, et al. Dietary glycemic index and load and the risk of type 2 diabetes: a systematic review and updated meta-analyses of prospective cohort studies. Nutrients 11 (2019): 1280.
- .Reynolds A, Mann J, Cummings J, et al. Carbohydrate quality and human health: a series of systematic reviews and meta-analyses. The Lancet 393 (2019): 434-445.
- Jayedi A, Soltani S, Jenkins D, et al. Dietary glycemic index, glycemic load, and chronic disease: an umbrella review of meta-analyses of prospective cohort studies. Critical reviews in food science and nutrition 62 (2022): 2460-2469.
- Luo J, Rizkalla SW, Alamowitch C, et al. Chronic consumption of short-chain fructooligosaccharides by healthy subjects decreased basal hepatic glucose production but had no effect on insulin-stimulated glucose metabolism. The American Journal of Clinical Nutrition 63 (1996): 939-945.
- Dewulf EM, Cani PD, Claus SP, et al. Insight into the prebiotic concept: lessons from an exploratory, double blind intervention study with inulin-type fructans in obese women. Gut 62 (2013): 1112.
- Alles MS, de Roos NM, Bakx JC, et al. Consumption of fructooligosaccharides does not favorably affect blood glucose and serum lipid concentrations in patients with type 2 diabetes. The American Journal of Clinical Nutrition 69 (1999): 64-69.
- Yamashita K, Kawai K, Itakura M. Effects of fructo-oligosaccharides on blood glucose and serum lipids in diabetic subjects. Nutrition Research 4 (1984): 961-966.
- Li L, Li P, Xu L. Assessing the effects of inulin-type fructan intake on body weight, blood glucose, and lipid profile: A systematic review and meta-analysis of randomized controlled trials. Food science & nutrition 9 (2021): 4598-4616.
- Baghi HB, Naghili B, Shanehbandi D, et al. Evaluation of a human gut-associated phage and gut dominant microbial phyla in the metabolic syndrome. Clinical Nutrition ESPEN 50 (2022): 133-137.
- Ahmad A, Yang W, Chen G, et al. Analysis of gut microbiota of obese individuals with type 2 diabetes and healthy individuals. PLOS ONE 14 (2020): e0226372.
- Naseri K, Saadati S, Ghaemi F, et al. The effects of probiotic and synbiotic supplementation on inflammation, oxidative stress, and circulating adiponectin and leptin concentration in subjects with prediabetes and type 2 diabetes mellitus: A GRADE-assessed systematic review, meta-analysis, and meta-regression of randomized clinical trials. European Journal of Nutrition 62(2023): 543-561.
- Magne F, Gotteland M, Gauthier L, et al. The firmicutes/bacteroidetes ratio: a relevant marker of gut dysbiosis in obese patients? Nutrients 12 (2020): 1474.
- Kato T, Kagawa M, Suda W, et al. Integrated Multi-Omics Analysis Reveals Differential Effects of Fructo-Oligosaccharides (FOS) Supplementation on the Human Gut Ecosystem. In International Journal of Molecular Sciences 23 (2022):11728.
- Das T, Jayasudha R, Chakravarthy S, et al. Alterations in the gut bacterial microbiome in people with type 2 diabetes mellitus and diabetic retinopathy. Scientific Reports 11 (2021): 2738.
- Méndez-Salazar EO, Ortiz-López MG, Granados-Silvestre MdlÁ, et al. Corrigendum: Altered Gut Microbiota and Compositional Changes in Firmicutes and Proteobacteria in Mexican Undernourished and Obese Children. Front. Microbiol 9 (2018): 2693.
- Shin N-R, Whon TW, Bae J-W. Proteobacteria: microbial signature of dysbiosis in gut microbiota. Trends in Biotechnology 33 (2015): 496-503.
- Ibrahim M, Anishetty S. A meta-metabolome network of carbohydrate metabolism: Interactions between gut microbiota and host. Biochemical Biophysical Research Communications 428 (2012): 278-284.
- Cani PD, Amar J, Iglesias MA, et al. Metabolic endotoxemia initiates obesity and insulin resistance. J Diabetes 56 (2007): 1761-1772.
- Salguero MV, Al-Obaide MAI, Singh R, et al. Dysbiosis of Gram-negative gut microbiota and the associated serum lipopolysaccharide exacerbates inflammation in type 2 diabetic patients with chronic kidney disease. Exp Ther Med 18 (2019): 3461-3469.
- Liu X, Mao B, Gu J, et al. Blautia—a new functional genus with potential probiotic properties? Gut Microbes 13 (2021): 1875796.
- Hosomi K, Saito M, Park J, et al. Oral administration of Blautia wexlerae ameliorates obesity and type 2 diabetes via metabolic remodeling of the gut microbiota. Nature Communications 13 (2022): 4477.
- Bouhnik Y, Vahedi K, Achour L, et al. Short-chain fructo-oligosaccharide administration dose-dependently increases fecal bifidobacteria in healthy humans. The Journal of nutrition 129 (1999): 113-116.
- Nogacka AM, Salazar N, Arboleya S, et al. In vitro evaluation of different prebiotics on the modulation of gut microbiota composition and function in morbid obese and normal-weight subjects: Int J Mol Sci 21 (2020): 906.
- Derrien M, Turroni F, Ventura M, et al. Insights into endogenous Bifidobacterium species in the human gut microbiota during adulthood. Trends in Microbiology 30 (2022): 940-947.
- Koh A, De Vadder F, Kovatcheva-Datchary P, et al. From Dietary Fiber to Host Physiology: Short-Chain Fatty Acids as Key Bacterial Metabolites. Cell 165 (2016): 1332-1345.
- Monteagudo-Mera A, Rastall RA, Gibson GR, et al. Adhesion mechanisms mediated by probiotics and prebiotics and their potential impact on human health. Applied microbiology and biotechnology 103 (2019): 6463-6472.
- Monteagudo-Mera A, Rastall RA, Gibson GR, et al. Adhesion mechanisms mediated by probiotics and prebiotics and their potential impact on human health. Applied Microbiology and Biotechnology 103 (2019): 6463-6472.
- Hou K, Wu Z-X, Chen X-Y, et al. Microbiota in health and diseases. Signal transduction and targeted therapy 7 (2022): 135.
- Marlicz W, Ostrowska L, ?oniewski I. Review paperThe role of gut microbiota in weight management by non-invasive interventions and bariatric surgery. Nutrition, Obesity & Metabolic Surgery 1 (2014): 20-29.
- Chen C, Zhang Y, Yao X, et al. Characterizations of the Gut Bacteriome, Mycobiome, and Virome in Patients with Osteoarthritis. Microbiology Spectrum 11 (2022): e01711-01722.
- Yang X, Zeng D, Li C, et al. Therapeutic potential and mechanism of functional oligosaccharides in inflammatory bowel disease: a review. Food Science and Human Wellness 12 (2023): 2135-2150.
- Huo J, Wu Z, Sun W, et al. Protective effects of natural polysaccharides on intestinal barrier injury: A review. Journal of agricultural and food chemistry 70 (2022): 711-735.
- Ai D, Pan H, Li X, et al. Association network analysis identifies enzymatic components of gut microbiota that significantly differ between colorectal cancer patients and healthy controls. PeerJ 7 (2019): e7315.
- Chérier D, Patin D, Blanot D, et al. The Biology of Colicin M and Its Orthologs. Antibiotics 10 (2021): 1109.
- Gozali C, Rukmana A. Potential of Colicin as an Antibacterial Agent in Escherichia coli. EKSAKTA: Berkala Ilmiah Bidang MIPA 24 (2023): 216-225.
- Matthewman C, Narin A, Huston H, et al. Systems to model the personalized aspects of microbiome health and gut dysbiosis. Molecular Aspects of Medicine 91 (2023): 101115.