Emergence of a Pathogenic Strain of COVID-19
Article Information
Shreyans Chatterjee1,4, Tathagata Dey2,4*, Smarajit Manna3,4
1Microbiology Department, St. Xavier’s College, Kolkata, India
2Computer Science Department, Government College of Engineering and Textile Technology, West Bengal, India
3Jagadis Bose National Science Talent Search, Kolkata, India
4Centre for Interdisciplinary Research and Education, Kolkata, India
*Corresponding Authors: Tathagata Dey, SBGP757, Santinagar, P.O. Sapuipara, P.S. Nischinda, Bally, Howrah, West Bengal, India
Received: 27 August 2020; Accepted: 28 September 2020; Published: 15 October 2020
Citation: Shreyans Chatterjee, Tathagata Dey, Smarajit Manna. Emergence of a Pathogenic Strain of COVID-19. Journal of Bioinformatics and Systems Biology 3 (2020): 081-091.
View / Download Pdf Share at FacebookAbstract
SARS-CoV-2 pandemic starting from Wuhan, China has now been spreading worldwide making the infection count more than 41 million. Within a short time span, many mutations are continuously occurring in the viral genome, be it point mutation or frameshift mutation. Scientists have been suggesting that, one of those numerous point mutations is becoming prevalent by replacing all the initial Wuhan strains of SARS-CoV-2. In this work, we have conducted a rigorous bio-informatic analyses and compared the properties of wild and mutant strains to find out the changes. Eventually, it is considered to be a more pathogenic and infective strain by our theoretical reports with a change in amino acid position number 614, which coincidentally converges with one or few publications mentioning emergence of new pathogenic D614G strain. Here we describe our approach to arrive at the conclusion.
Keywords
Mutation; SARS-CoV-2; D614G; Pathogenicity; 𝑞𝑅; Polar plot; Protein
Mutation articles, SARS-CoV-2 articles; D614G articles; Pathogenicity articles; q_R articles; Polar plot articles; Protein articles
Mutation articles Mutation Research articles Mutation review articles Mutation PubMed articles Mutation PubMed Central articles Mutation 2023 articles Mutation 2024 articles Mutation Scopus articles Mutation impact factor journals Mutation Scopus journals Mutation PubMed journals Mutation medical journals Mutation free journals Mutation best journals Mutation top journals Mutation free medical journals Mutation famous journals Mutation Google Scholar indexed journals SARS-CoV-2 articles SARS-CoV-2 Research articles SARS-CoV-2 review articles SARS-CoV-2 PubMed articles SARS-CoV-2 PubMed Central articles SARS-CoV-2 2023 articles SARS-CoV-2 2024 articles SARS-CoV-2 Scopus articles SARS-CoV-2 impact factor journals SARS-CoV-2 Scopus journals SARS-CoV-2 PubMed journals SARS-CoV-2 medical journals SARS-CoV-2 free journals SARS-CoV-2 best journals SARS-CoV-2 top journals SARS-CoV-2 free medical journals SARS-CoV-2 famous journals SARS-CoV-2 Google Scholar indexed journals D614G articles D614G Research articles D614G review articles D614G PubMed articles D614G PubMed Central articles D614G 2023 articles D614G 2024 articles D614G Scopus articles D614G impact factor journals D614G Scopus journals D614G PubMed journals D614G medical journals D614G free journals D614G best journals D614G top journals D614G free medical journals D614G famous journals D614G Google Scholar indexed journals Pathogenicity articles Pathogenicity Research articles Pathogenicity review articles Pathogenicity PubMed articles Pathogenicity PubMed Central articles Pathogenicity 2023 articles Pathogenicity 2024 articles Pathogenicity Scopus articles Pathogenicity impact factor journals Pathogenicity Scopus journals Pathogenicity PubMed journals Pathogenicity medical journals Pathogenicity free journals Pathogenicity best journals Pathogenicity top journals Pathogenicity free medical journals Pathogenicity famous journals Pathogenicity Google Scholar indexed journals ?? articles ?? Research articles ?? review articles ?? PubMed articles ?? PubMed Central articles ?? 2023 articles ?? 2024 articles ?? Scopus articles ?? impact factor journals ?? Scopus journals ?? PubMed journals ?? medical journals ?? free journals ?? best journals ?? top journals ?? free medical journals ?? famous journals ?? Google Scholar indexed journals Polar plot articles Polar plot Research articles Polar plot review articles Polar plot PubMed articles Polar plot PubMed Central articles Polar plot 2023 articles Polar plot 2024 articles Polar plot Scopus articles Polar plot impact factor journals Polar plot Scopus journals Polar plot PubMed journals Polar plot medical journals Polar plot free journals Polar plot best journals Polar plot top journals Polar plot free medical journals Polar plot famous journals Polar plot Google Scholar indexed journals Protein articles Protein Research articles Protein review articles Protein PubMed articles Protein PubMed Central articles Protein 2023 articles Protein 2024 articles Protein Scopus articles Protein impact factor journals Protein Scopus journals Protein PubMed journals Protein medical journals Protein free journals Protein best journals Protein top journals Protein free medical journals Protein famous journals Protein Google Scholar indexed journals RNA viruses articles RNA viruses Research articles RNA viruses review articles RNA viruses PubMed articles RNA viruses PubMed Central articles RNA viruses 2023 articles RNA viruses 2024 articles RNA viruses Scopus articles RNA viruses impact factor journals RNA viruses Scopus journals RNA viruses PubMed journals RNA viruses medical journals RNA viruses free journals RNA viruses best journals RNA viruses top journals RNA viruses free medical journals RNA viruses famous journals RNA viruses Google Scholar indexed journals ACE2 articles ACE2 Research articles ACE2 review articles ACE2 PubMed articles ACE2 PubMed Central articles ACE2 2023 articles ACE2 2024 articles ACE2 Scopus articles ACE2 impact factor journals ACE2 Scopus journals ACE2 PubMed journals ACE2 medical journals ACE2 free journals ACE2 best journals ACE2 top journals ACE2 free medical journals ACE2 famous journals ACE2 Google Scholar indexed journals COVID-19 articles COVID-19 Research articles COVID-19 review articles COVID-19 PubMed articles COVID-19 PubMed Central articles COVID-19 2023 articles COVID-19 2024 articles COVID-19 Scopus articles COVID-19 impact factor journals COVID-19 Scopus journals COVID-19 PubMed journals COVID-19 medical journals COVID-19 free journals COVID-19 best journals COVID-19 top journals COVID-19 free medical journals COVID-19 famous journals COVID-19 Google Scholar indexed journals
Article Details
1. Introduction
SARS-CoV-2 or COVID-19 being a pandemic (declared by W.H.O. on 11th March, 2020) has already spread to 211 countries worldwide, making the total count of infection cases to 7,273,958 with 413,372 deaths as on 11th June, 2020 [1]. SARS-CoV-2, commonly called as COVID-19, is an enveloped, single-stranded positive sense RNA virus, which falls under the family of Coronaviridae and has most likely jumped the species boundary [2] as other coronaviruses likely did. The Reproduction Number (R0) of COVID-19 is approximately 3.28 [3], so it is highly contagious. It spreads via respiratory droplets and contact routes, infecting the upper and lower respiratory tracts which increases its potential to spread in a community [4]. People infected by this virus show a variety of symptoms with a median incubation period of 5.1 days [5].
It has been observed that unlike other RNA viruses, SARS-CoV-2 mutates at a slower rate [6]. Recently a prominent mutation has been observed in the spike glycoprotein of many reported viral sequences [7]. This spike glycoprotein helps the virus to attach with the host cell receptor, ACE2 (Angiotensin Converting Enzyme 2) and TMPRSS2 [8]. Point mutations in COVID-19 spike glycoprotein can lead to change in its infectivity and may also require extensive studies for sustainable vaccine designing [7, 9].
In this article we have analyzed the above-mentioned mutations in order to find out if any particular mutation is predominant. We have also discussed through various bioinformatics tools probable effects on the biochemical nature of the protein due to the predominant mutation and how it can affect the host receptor cells. Our analysis consists of mutation analysis, checking surface exposure and thermodynamic stability. These give us an interpretation about how the mutated protein is going to interact with the receptor with respect to wild protein.
2. Methodology
The flowchart of the work procedure along with their objectives stand as the following:
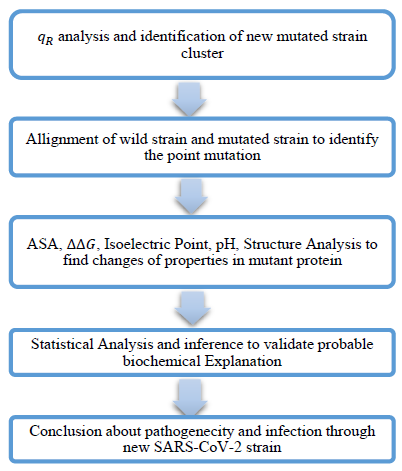
We used 2D Polar Co-ordinate Representation of Amino Acid Sequences and value characterization [10] to study the COVID-19 gene sequences. In this method, we assign angles to each amino acid depending upon their relative hydrophobicity indices and move one unit in the respective assigned direction. We assume the graph to contain unit masses at each co-ordinate and we determine the distance of center of mass of the graph from the origin, which is defined as the Quotient Radius or value of the graph. This value is found to be a characteristic value of a sequence [10].
We investigated the spike glycoprotein sequences of COVID-19 through graph plotting, qR value characterization and distribution plots. We have used MEGA-X to align the sequences and find out the mutational changes in it [11]. We used iMutant 3.0 to find out the stability changes in the mutated sequence from the first reported Wuhan sequence due to single point mutations [12]. We have used SABLE software [13] and RaptorX [14] to identify the solvent accessibility and secondary structure of the individual amino acids present in the protein sequences. We used Phyre2 [15] to generate pdb format for the spike protein sequences and also used UCSF Chimera [16] to visualize them.
Using the qRplot we found out the dominant mutations in the recently reported strains of COVID-19. Sequences having identical values indicate that they have identical qR amino acid sequences while sequences with close qR values have one or more changes in sequences [10]. The mutations can be observed from the difference in the qR values of Wuhan sequence and other sequences. We can obtain the value from
where qRi represents the qR value of sequences other than original Wuhan sequence (YP009724390).
The solvent accessibility of individual amino acids indicates whether the particular amino acid is exposed to the solvent or buried inside the core of the protein or whether it is located in an intermediate zone. Amino acids that are more exposed at the surface have more probability in either directly taking part or catalyzing interactions between viral protein and host receptors. This Average Solvent Accessibility (ASA) profile is determined by using SABLE Software and RaptorX [14].
The change in Gibb’s free energy (ΔG) for a protein is an important factor as it is directly related to protein folding and protein stability. We calculated the ΔΔG value for a single point mutated protein using iMutant 3.0 [12]. Where,
If , then the protein is less stable while if then the protein is relatively more stable.
In case of SARS-COV-2, the spike glycoprotein uses ACE2 (Angiotensin Converting Enzyme 2) as a receptor for attachment to the host cell. Then the host serine-protease, TMPRSS2 (Transmembrane Serine Protease 2) does S (Spike) priming allowing fusion between viral and host cell membrane and helps in the viral entry inside the cell. So, by analyzing how the spike glycoprotein attaches with ACE2 we can understand how mutation has changed the infectivity of the new strain. ACE 2 is mainly attached to the cell membrane of lung type II alveolar cells. The pH of a healthy lung ranges between 7.38 to 7.42 [17]. All our calculations are done taking the average pH 7.40.
3. Results
3.1 qRCharacterization Analysis
We have analyzed the values of the 46 sequences of Europe, 2052 sequence of North America and 14 sequences of India of Spike Glycoprotein of SARS-CoV-2 present in NCBI Database as on 9th May, 2020 [18].
In Europe out of those 46 sequences, 11 sequences had qR value as around 30.16105055, which is equal to Original Wuhan sequence qR value (YP009724390). So, we expect no mutations to take place in these sequences. Whereas in all other sequences, the was different. However, one significant cluster can be made amongst them, where 16 sequences of the rest had same value as 29.76636445. This value (29.76636445) is first observed in a sequence from Spain, collected on 9th March, 2020 (QIU78707).
In case of North America, there was much abundance of sequences, the number of sequences being 2052. We computed all of them through our characterization analysis. 601 of them had qR value as 30.16105055. So, it implies that they were same as initial wild strains collected from Wuhan. Amongst the rest of North American sequences, we again found a large cluster, containing 1067 sequences and having the same qR value as of European mutated cluster, being 29.76636445. The said American cluster first Δ appeared in database on 11th March 2020, collected from San Diego County, California, USA (QIK50427).
From India, there were 14 sequences uploaded in database, which we computed through qR characterization. 5 of them had original Wuhan Strain’s qR value, while 4 out of the 14 had this mutated qR value. The mutated sequence first appeared on 11th March, 2020 (QJF77858). The mutated strain can be identified from. ΔqR=-0.3946861
A very similar observation of this particular cluster of qR values in USA was mentioned in one of our earlier papers [7]. From the obtained dataset we see that this mutated strain (of qR value 29.76636445) is abundant in whole of the world, irrespective of demography and also the count of such sequences is more than original wild strain in most of the cases. This observation tells us about the importance of this mutation. We plotted the 2D polar graphs of qR values for spike glycoprotein of Wuhan strain and the mutated strains in Figure 1.
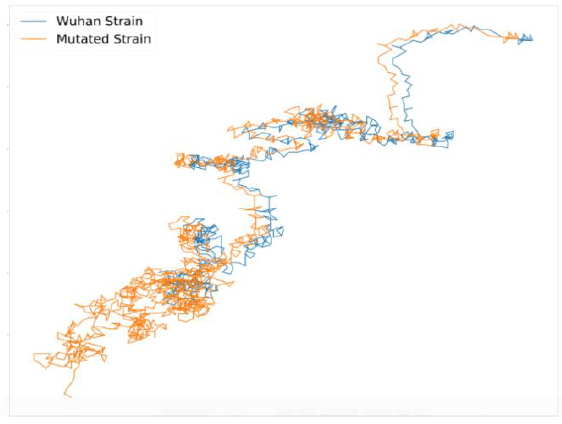
Figure 1: 2D Polar Plot of Wild strain (in blue) and Mutant strain (in red) of SARS-CoV-2 Spike Glycoprotein.
It can be observed from Figure 1 that both of them shows similar graphs except that the mutated sequence has formed a parallel shift leftwards after an initial part of the sequence. From our principle of plotting, this indicates an occurrence of point mutation in its peptide sequence.
3.2 Alignment
We used MEGA-X to align the sequences. The mutation was found at the position 614 of the peptide sequence where an Aspartic Acid (D) was replaced by Glycine (G). This mutation was previously reported as D614G [19, 20].
3.3 Amino Acid Solvent Accessibility
The amino acid solvent accessibility was calculated simultaneously for the initial Wuhan sequence and the D614G mutated sequence. The results are shown in Table 1.
SEQUENCE |
POSITION: 614 |
||
Amino Acid |
Accessibility Nature |
Value |
|
Wuhan (Wild) |
D (Aspartic Acid) |
Intermediate |
0.305 |
D614G (Mutant) |
G (Glycine) |
Exposed |
0.337 |
Table 1: Solvent Accessibility Table for Wild and Mutant Strain.
Glycine is more exposed towards the solvent than Aspartic acid, though between them, Aspartic acid is more water soluble (polar nature) and should have a higher solvent accessibility. This anomalous behavior of Aspartic acid and Glycine may be related to its function in the host cells.
3.4 Calculation of ΔΔG Value
The ΔΔG values were bioinformatically calculated for the Wuhan sequence and the Mutated sequence. The result obtained is shown in Table 2.
pH |
Temperature |
ΔΔG = ΔGmutated - ΔGWuhan |
7.40 |
298.0 K |
-0.94 Kcal/mol |
Table 2: ΔΔG value of wild and mutant strain.
The decrease in the ΔΔG value due to the single point mutation at site 614 from Aspartic Acid to Glycine indicates a large decrease in stability of the mutated protein. Since, the stability of a protein is somewhat balanced with its activity [21], there is a large probability of alteration in the Spike-protein interactions and activities due to the mentioned ΔΔG change.
4. Discussions
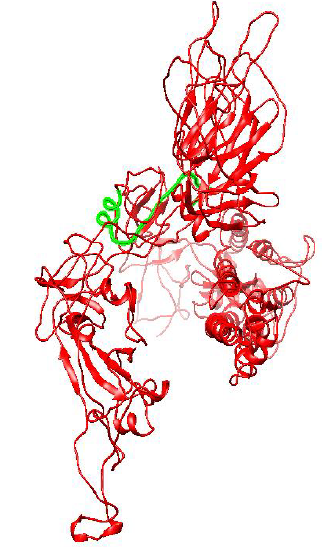
Figure 2: Protein Structure of Spike Glycoprotein of Wuhan (Wild) type strain of SARS-CoV-2.

Figure 3: Protein structure of Spike Glycoprotein of Mutant D614G strain of SARS-CoV-2.
Figure 2 and 3 respectively shows parts of the spike glycoprotein of initial Wuhan sequence (Y009724390) and D614G strain obtained from UCSF Chimera from the same perspective. The highlighted blue region is the aspartic acid in the sequence of Wuhan strain (GTNTSNQVAVLYQDVNCTEVPVAI) and in the case of D614G strain it is the Glycine amino acid (GTNTSNQVAVLYQGVNCTEVPVAI). A difference in the structure of the two proteins can be observed within the circled region in the figures. This difference is probably caused due to the D to G mutation in the sequence.
We can write the reaction between the spike glycoprotein receptor of COVID-19 and host ACE2 as the following
a[spike glycoprotein]+b[ACE2]->b[spike glycoprotein-ACE2 complex] (1)
That is, a moles of spike glycoprotein reacting with b moles of ACE2 form b moles of the complex, since the number of ACE2 receptors is a constant in the cell, that is, the number of ACE2 is not a function of number of viral spike protein. The number of complexes formed between host receptor and viral spike protein is totally dependent on the concentration of ACE2 in the cell which remains constant for each healthy individual.
Aspartic acid (D), located at a C-coil in the Wuhan strain, on mutation to Glycine (G) resulted in a ΔΔG value of -0.94 indicating a greater instability. Most mutations leading to novel functions are destabilizing [21]. A destabilizing mutation to Glycine changes the biochemical nature of the protein at that site by making Glycine more solvent accessible and making the mutation site more prone to novel chemical reactions [19]; finally, increasing its attachment potential with ACE2 and ultimately increasing its stability. We have also graphically plotted the distribution of this mutation versus date of collection which is shown in Figure 4.
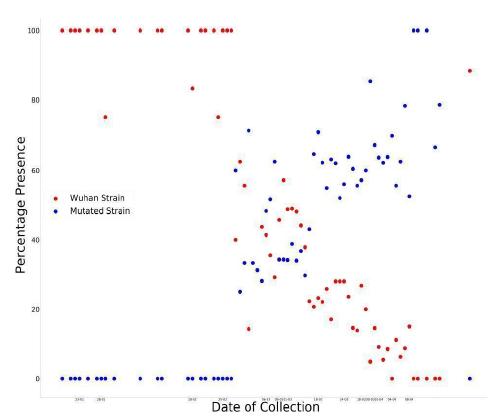
Figure 4: Graph of percentage of wild strains and mutant strains in collected sequences with respect to time.
In Figure 4, a point represents that on the day , if there were 100 collected sequences, then no. of sequences of that strain was The plot resembles the ideal graphical plot of concentration versus time of reactants and products. So, it can be mathematically written as,
The relative rate of formation of D614G strain is more prominent than any other mutation in the spike glycoprotein sequence. The more affinity towards the directed formation of the said mutation implies that this point mutation helps the virus to sustain itself. It also indicates that the D614G strain can attach more with ACE2 receptor and hence propagate, thus converging with our Bio-Chemical inference. Previously we saw that the mutation makes the new strain comparatively less stable; from these data we find out that the D614G strain is increasing in number, i.e. it has a higher infection rate; this is plausible if we consider that the region surrounding the mutation-site becomes a hotspot for chemical reaction favoring a stronger attachment with ACE2. This evidently explains the high surge in percentage of presence for this strain. The D614G strain is increasing in quantity in nature in due course of time, while the initial Wuhan strain is becoming obsolete.
So, all these data and values indicate a rightward shift in the chemical reaction (1). A more favorable formation of the complex simultaneously implies more infection and more spread of the D614G strain of COVID-19. The graph below shows the superimposed plot of presence of the studied mutated strains and reported infection in various states of USA. We can see that a surge in the number of reported sequences is proportional to the increase in infections.
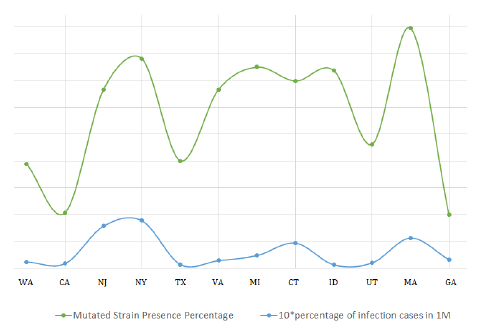
Figure 5: Superimposition of infection rate in states vs abundance ofD614G strain.
Also, if we plot the case fatality ratio vs the percentage presence of mutant D614G strain, again a very similar coherent pattern is found which again supports our theory. The graph is shown in Figure 6.
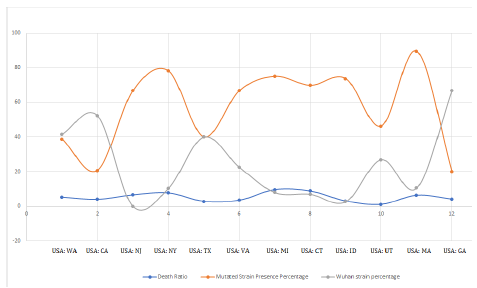
Figure 6: Superimposition of wild and mutant strain presence vs death ratio in various states.
In Figure 6, the orange curve represents the percentage presence of D614G strains and Gray curve shows percentage presence of Wuhan strain while the blue curve is the Case Fatality Ratio. Case Fatality Ratio is defined as the number of fatal cases of infection amongst total number of infected patients. We see the mutant strain abundance and case fatality ratio are exactly coherent and run with almost zero phase difference. That is, case fatality ratio amongst the infected people is high when the state has more number of D614G strains. The more is the number of D614G strains, the more is the chances of people being infected with this strain which eventually converges with higher death rate. This may be interpreted as its more pathogenic nature. Also, these mutated strains were first collected from various continents from March 9-11, 2020 onwards, which also satisfies the increasing (polynomial increase) counts from mid of March, 2020.
5. Conclusion
So, it is evident that a mutated strain D614G has been formed and is becoming quite dominant with respect to time replacing the initial Wuhan sequence. This new strain is probably more infectious due to its change in biochemical properties which increases its attachment potential with the ACE2 receptor. This mutation helps the virus to propagate, but is deadly for humans as it increases the rate of infection.
Author Contributions
Shreyans Chatterjee did the bioinformatic analysis of the sequences. Tathagata Dey and Smarajit Manna collected data and performed statistical analysis.
Declaration of Competing Interest
The authors declare no conflict of interest.
Acknowledgement
We would like to thank Prof. Subhash C. Basak and Dr. Ashesh Nandy for their valuable advices and comments during the course of this work.
Funding
This research did not receive any specific grant from funding agencies in the public, commercial or not-for-profit sectors.
References
- Coronavirus disease (COVID-19). Situation Reports. WHO (2020).
- Zhang YZ, Edward C Holmes. A Genomic Perspective on the Origin and Emergence of SARS-CoV-2. Cell 181 (2020): 223-227.
- Liu Y, Albert A Gayle, Wilder-Smith A, et al. The reproductive number of COVID-19 is higher compared to SARS Coronavirus. Journal of Travel Medicine 27 (2020).
- Bartolome S. Life cycle of a coronavirus: How respiratory illnesses harm the body. UT Southwestern Medical Center (2020).
- Lauer SA, Grantz KH, Bi Qifang, et al. The Incubation Period of Coronavirus Disease 2019 (COVID-19) From Publicly Reported Confirmed Cases: Estimation and Application. Annals of Internal Medicine (2020).
- Sanjuán R, Domingo-Calap P. Mechanisms of Viral Mutation, Cellular and Molecular Life Sciences. CMLS 73 (2016): 4433-4448.
- Dey T, Chatterjee S, Manna S, et al. New Computational Analysis to Identify the Mutational Changes in SARS-CoV-2, MOL2NET, International Conference on Multidisciplinary Sciences USINEWS-04 (2020).
- Hoffmann M, Kleine-Weber H, Schroeder S, et al. SARS-CoV-2 Cell Entry Depends on ACE2 and TMPRSS2 and Is Blocked by a Clinically Proven Protease Inhibitor. Cell 181 (2020): 271-280.
- Dawood AA. Mutated COVID-19 May Foretells Mankind in a Great Risk in the Future. New Microbes and New Infections 35 (2020).
- Dey T, Biswas S, Chatterjee S, et al. 2D Polar Co-ordinate Representation of Amino Acid Sequences With some applications to Ebola virus, SARS and SARS-CoV-2 (COVID-19), MOL2NET, International Conference on Multidisciplinary Sciences USINEWS-04, UMN, Duluth, USA (2020).
- Kumar S, Stecher G, Li M, et al. MEGA X: Molecular Evolutionary Genetics Analysis across computing platforms. Mol Bio Evol 35 (2018): 1547-1549.
- Capriotti E, Fariselli P, Casadio R. I-Mutant2.0: predicting stability changes upon mutation from the protein sequence or structure. Nucleic Acids Res 33 (2005).
- Accurate sequence-based prediction of relative Solvent AccessiBiLitiEs, secondary structures and transmembrane domains for proteins of unknown structure. Sable (2020).
- Källberg M, Wang H, Wang S, et al. Template-based protein structure modeling using the RaptorX web server. Nature Protocol 7 (2012): 1511-1522.
- Kelley LA, Mezulis S, Yates CM, et al. The Phyre2 web portal for protein modeling, prediction and analysis. Nature Protocols 10 (2015): 845-858.
- Pettersen EF, Goddard TD, Huang CC, et al. UCSF Chimera-A Visualization System for Exploratory Research and Analysis. Journal of Computational Chemistry 25 (2004).
- Giorno F. The pH level of Healthy Lungs. Livestrong 27 (2011).
- National Center for Biotechnology Information (NCBI).
- Bhattacharyya C, Das C, Ghosh A, et al. Global Spread of SARS-CoV-2 Subtype with Spike Protein Mutation D614G is Shaped by Human Genomic Variations that Regulate Expression of TMPRSS2 and MX1 Gene. bioRxiv (2020).
- Korber B, Fischer WM, Gnanakaran S, et al. Spike mutation pipeline reveals the emergence of a more transmissible form of SARS-CoV-2. bioRxiv (2020).
- Tokuriki N, Stricher F, Serrano L, et al. How Protein Stability and New Functions Trade Off. PLoS Computational Biology (2008).